Genomic prediction of IQ is modern snake oil
Predicting IQ from genetics is inaccurate, confounded, and sows confusion about genetic research.
Update: After this post, Kian Sadeghi at Nucleus wrote about their IQ prediction platform and discussed some of the criticism.
Genetic testing companies have started to venture into behavioral traits, with predictable controversy. The latest, a startup called Nucleus (funded in part by Alexis Ohanian and Peter Thiel), recently launched a closed beta for genetic prediction of IQ. The launch started off oddly, debuting on a tech podcast that also featured financial criminal Martin Shkreli (there to discuss a Trump-branded cryptocurrency project), and immediately veered into discussion of embryo selection — a product Nucleus does not offer. The actual product, Nucleus IQ, a genetic predictor for adults which currently only exists publicly as a sign-up screen, drew immediate criticism from biologists (here, here, here, and by me) as well as the allusions to Gattaca and eugenics that typically swirl around this topic.
Naturally, the founder of Nucleus responded by doubling-down. Penning a long post on the X social network appealing to “information access and liberty” with dire warnings that “the suppression of knowledge splinters society, catalyzes misinformation, and undermines our ability to understand ourselves and each other”, a series of “We believe …” proclamations, a tag of OpenAI CEO Sam Altman, the promise of “a golden era in understanding our own biology”, and, finally, an invitation to “be part of the future, today?”. As some sharply observed, it all felt a bit orchestrated: an over-the-top edgy release to gin up a controversy followed by an over-the-top response about making the world a better place (or maybe to pick up the phone and start dialing, depending on the target audience).
Notably absent from this manifesto was any evidence that their predictor is accurate, or even how it is calculated. Apparently in the golden era of understanding we do not need to concern ourselves with details like “does it actually work?”. So let’s see what these tests really do and how much understanding they can provide.
What is genomic prediction?
It’s good to start from the very beginning. Like I tell my kids, when two people love each other very much, they go to a special store and buy a baby that gets half it’s DNA from one parent and half from the other. When that baby grows up, it can choose to participate in a Genome-Wide Association Study (GWAS), where geneticists combine it’s DNA with that of hundreds of thousands of other study participants and, for each genetic variant, ask if it is more common in those who have a certain trait than those who don’t. This is called a genetic association and each GWAS estimates millions of them. Even though these associations are genetic, they are still mostly not causal, for three fundamental reasons:
Variants that are nearby in the genome are correlated, so any causal variant will often have many other correlated non-causal associations around it. In truth, these studies do not identify variants, but rather large associated regions with many correlated associations of which only one or two are truly causal. While it’s possible to shortlist the one mutation that’s likely to actually be doing something with various computational tricks, ultimately you need confirmation from real experiments or interventions. And while hundreds of thousands of GWAS have been identified, only a tiny fraction of these have actually been validated experimentally.
Variants are inherited from parents (and grand-parents, and so on), so if a variant is actually doing its something in your parents (or uncles, or grand-parents, etc) and that has an impact on your phenotype, it will naively look like it’s doing something in you. Since most GWAS do not include genotyped parents, they will pick these variants up as associations even when they no longer do anything. In fact, such a variant doesn’t even have to do anything to the trait at all if it does something to a different trait that people partner up on (aka cross-trait assortative mating). If tall people marry thin people, then variants increasing height and variants decreasing weight will become correlated in the offspring, and come up as associations in GWAS of height or weight. These two processes can combine to become a substantial cultural mechanism: a variant influences the trait in one generation, this in turn influences the traits in the children, who then find partners with similar traits, pass those down again to their kids, and so on.
Even variants that do nothing at all in anyone can still be picked up as associations if they are incidentally correlated with the phenotype through population stratification or other technical confounders. GWAS has gotten pretty good at ruling these out but it’s not perfect, especially for phenotypes that exhibit complex cultural stratification over many generations (foreshadowing).
It turns out we don’t need causality just to make a prediction. If we simply sum up all the genetic variants you carry, weighted by the strength and direction of their GWAS association, we can get an estimate of your total polygenic score. This score will, of course, include both the causal and non-causal variants, the variants having influence in prior generations, the false positives due to stratification, and so on. When this is done in independent samples, it tends to correlate with the target trait at a small but statistically significant level. As a result, much of GWAS has been oriented towards bigger and bigger studies so that the weights for these scores can be estimated with more accuracy. Almost all of the data is generated by academic institutions and is public, and you can go to the PGS Catalog or the PGI Repository or the PRS Knowledge Base (we love rebranding these scores, don’t we folks?) and download the weights needed to compute these scores right now (yes, even scores for IQ). Companies like Nucleus are sequencing the genetic variants of their clients, (most likely) downloading these public weights, computing the weighted sum, and putting it in a nice web interface. That’s about it.
But I was told there would be AI?
Sam Altman can mute his notifications, there is very little AI involved. The GWAS to discover associations is simply computing a lot of ordinary regressions. Then more (typically penalized) regressions are applied to shrink the weights to improve their joint prediction accuracy. If you squint, there is some AI that can go into the basic variant sequencing and calling steps, and there is ongoing research in how to incorporate AI to improve these models further. But at at it’s heart the approach is as simple as described above and the final score is really just a weighted sum or very close to it.
How accurate is genomic prediction in general?
The short answer is: it’s not very accurate. Genetic variation is associated with essentially all traits, but only a little bit! And this is especially true for the common genetic variants that go into most GWAS to date.
As a representative example, [Tanigawa et al. 2022] built and evaluated polygenic scores for 813 traits in the UK Biobank using ~269,000 White British individuals. In their analysis, the average polygenic score had a predictive accuracy of 1.6% (R2 for continuous traits, pseudo-R2 for binary traits). For comparison, a non-genetic model with just age, sex, and measures of fine-scale ancestry achieved an average accuracy of 7.5%. So, at the moment, the typical genetic score is telling you much less than age, sex, and where you live.
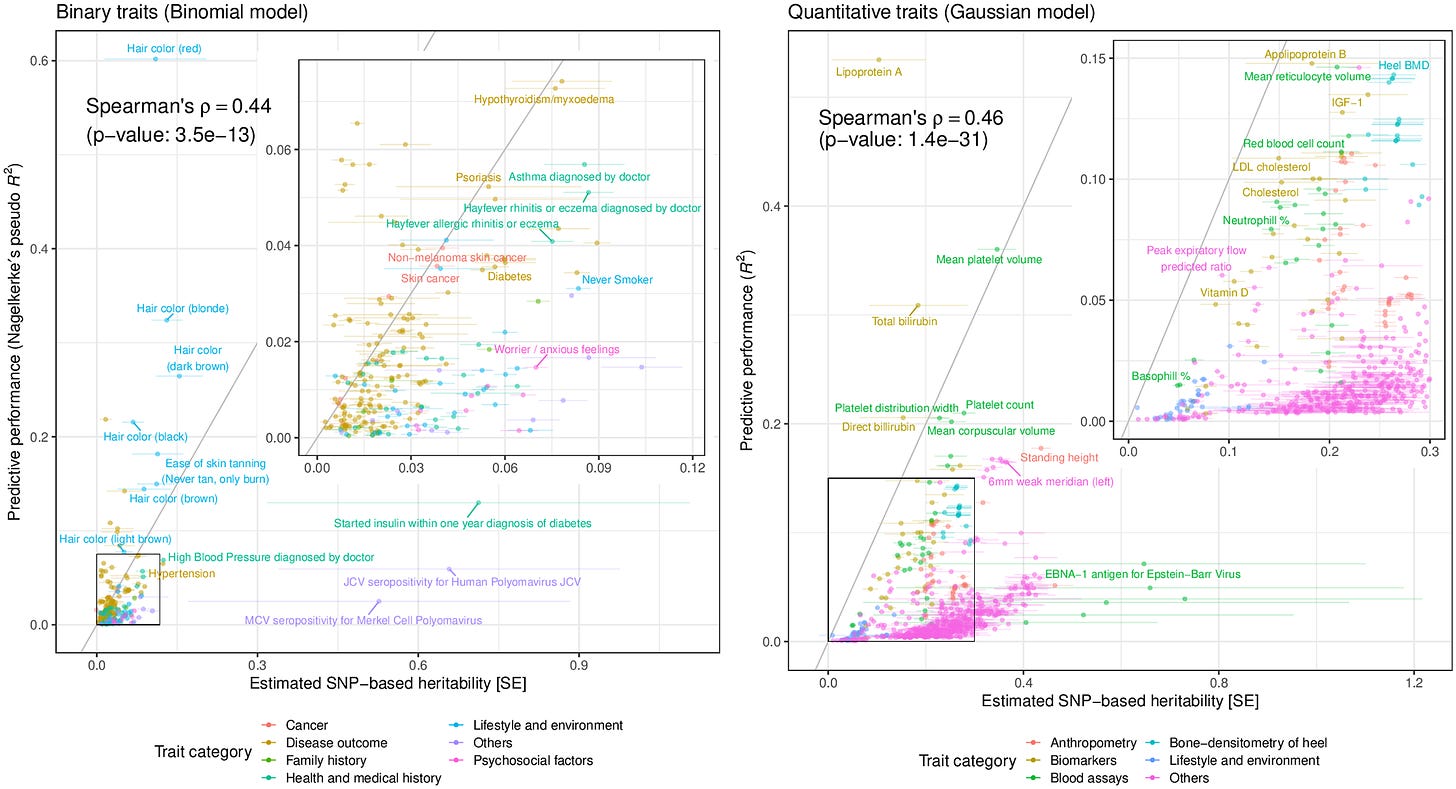
As we can see in the figure above, there are a handful of outlier traits with prediction accuracy > 20% — hair color, blood counts, or height — and a larger number of traits with modest but potentially useful predictions in the 5-15% range — cholesterol, some autoimmune conditions, cancer, etc. GWAS that specifically set out to ascertain disease cases have found similar results. For prostate cancer, a baseline model with age and family history achieves an AUC of 0.78, whereas adding a polygenic score bumps that up to 0.84 [Conti et al. 2021]. For breast cancer, a baseline model with age and risk factors had a C-statistic of 0.56, while adding a polygenic score bumps this up to 0.65 [Lakeman et al. 2020]. Modest, but potentially useful, especially when combined with existing risk factors in a comprehensive model.
As GWAS sample sizes increase and the associations are estimated with higher precision, the resulting polygenic score accuracy will likely increase too. But with the magic of statistics we can already put an upper bound on what the best prediction accuracy can be. This is the estimated “SNP-heritability” (shown on the x-axis in the figure above), or total variance in the trait explained by all genotyped variants (and any variants they are correlated with). In [Tanigawa et al. 2022], the average SNP heritability was 17% (and 23% for continuous traits), indicating that even with all of the training data in the world, a predictor built from common variant GWAS will end up in the modest but potentially useful range for the typical trait.
To be clear, there are some significant improvements over the existing clinical models and they are likely to be worth the relatively low cost of genotyping. But, for multifactorial traits, genetics is just one small risk factor of very many, with the vast majority of variation in risk simply unexplained (aka bad luck).
IQ prediction accuracy is low and will stay low
Given the historic controversies around cognitive traits, one might be surprised to learn that the genetic influences have been quite well studied. Most recently, [Chen et al. 2023] conducted a large-scale GWAS and rare variant analysis of multiple traits related to cognitive function in ~450,000 people in the UK Biobank. These traits included an actual short-form IQ test (which they call “Verbal Numeric Reasoning” and prior studies have called “Fluid IQ”), a reaction time test, and a measure of educational attainment. This study is particularly relevant to Nucleus because it’s one of the largest analyses of rare variants to date. Rare variants are the proposed added value of whole-genome sequencing over more conventional (and less edgy) genetic testing companies like 23andme, which only collect GWAS-level data.
What did they find? A common polygenic score explained 2.88% of the variance in IQ scores in held-out samples; highly statistically significant but modest and in-line with the above findings for typical traits. A rare gene burden score, constructed using rare mutations in the coding region of genes associated with cognitive function, explained 0.17% of the variance in IQ score. No, that is not a typo: less than one fifth of one percent! Thus the rare burden predictor — the value proposition for companies like Nucleus — is essentially a rounding error in terms of prediction. Projecting what the rare variant contribution will look like in the future is difficult, but there are “SNP-heritability”-like quantities that can be estimated for rare coding variants, and they indicate that the contribution of rare burden will be small (~1% on average [Weiner, Nadig et al. 2023]).
An alternative approach is to use polygenic scores developed for other traits related to IQ that are more easily available, such as educational attainment. Since education has a causal effect on IQ, a genetic score that predicts educational attainment will also predict IQ by proxy. The largest GWAS of educational attainment gathered data from an absolutely massive ~3 million individuals [Okbay et al. 2022] to estimate GWAS effects with extremely high accuracy, and this score was able to explain ~6% of IQ (or what they call “cognitive performance”). This is likely where predictor accuracy will stand for some time, as sample size has a non-linear relationship with accuracy and much larger studies are needed for any noticeable improvements.
In short, the best currently available genetic predictor explains ~2.8% (about 2.5 IQ points), and even an alternative proxy predictor based on a much larger study of education explains ~6% (about 3.7 IQ points). The total GWAS SNP-heritability of IQ is ~19% [Savage et al. 2018], so even assuming a perfect polygenic score could eventually be constructed, that score would explain about 6.5 IQ points. I’ve provided a visualization of this level of accuracy in the figures below, though you can also just close your eyes and imagine random noise. For context, the average difference in IQ scores (or, more specifically, an extracted general factor g) from the same individual taken ~30 days apart is ~8 points [Fawns-Ritchie et al. 2020]. So even at the maximum hypothetical accuracy, a genetic score will explain less than the test-retest error bar.
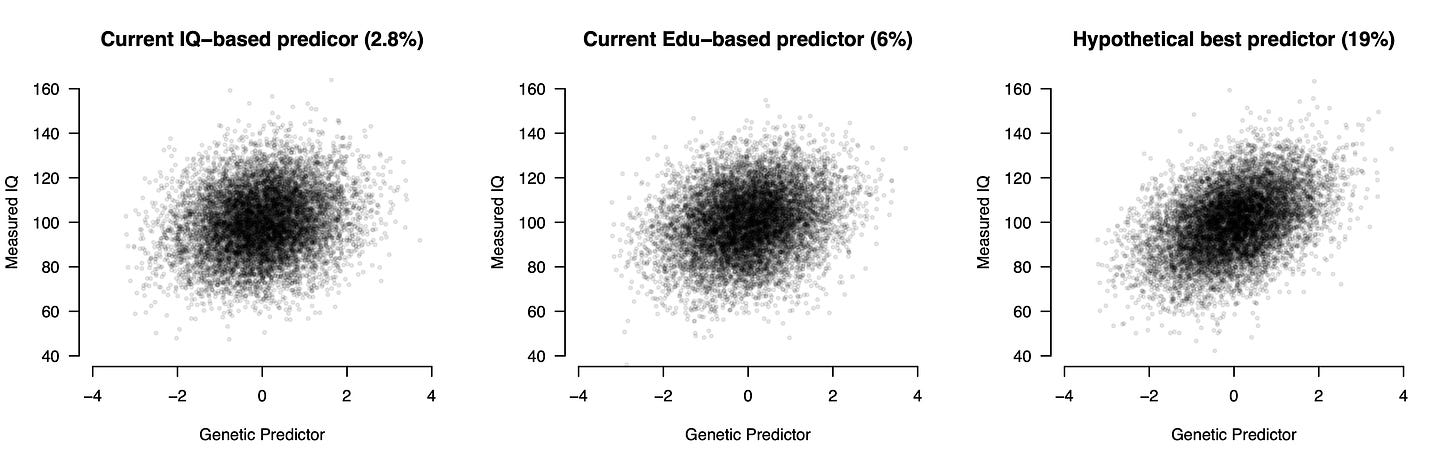
You don’t even need to take my word for it. To accompany their GWAS of education, the authors (part of a major Social Science Genetics Consortium) issued a detailed FAQ explaining that their score cannot be used to predict individual educational outcomes (but may be useful in other settings). The FAQ contains the following warning, which seems particularly relevant:
The results of SSGAC studies have sometimes been used by online platforms, including some companies, to predict individual outcomes. We recognize that returning individual genomic “results” can be a fun way to engage people in research and other projects and to feed or stoke their interest in genomics. But it is important that participants/users understand that these individual results are not meaningful predictions and should be regarded essentially as entertainment. Failure to make this point clear risks sowing confusion and undermining trust in genetics research. [bold mine]
A large component of predicted IQ is not causal
As noted above, one of the ways non-causal correlations sneak into the genetic predictor is through intergenerational correlation of phenotypes. While for most traits this type of confounding appears to be negligible, for traits related to education and socioeconomic status it is substantial. A simple way to appreciate this is to look at how a genetic predictor of education changes when adding parent education into the model: when [Lee et al. 2018] added parental education to a model with a genetic predictor of educational attainment, the accuracy of the genetic predictor dropped from 12% to 5%. Thus, you can already approximate the majority of your genetic score simply by asking your parents how much schooling they received. Indeed, parental education alone achieved a prediction accuracy of >20% for educational attainment in [Lee et al. 2018], greater than what could be explained by all GWAS variants.
When genetic data from families is available, one can also quantify the total fraction of SNP-heritability that is acting “directly” (i.e. in the individual1) versus that which is correlated with “indirect” associations through parents, family members, and stratification. These sophisticated methods confirm what was observed crudely with polygenic scores: a large proportion of the genetic associations with IQ-related phenotypes are simply non-causal. For educational attainment, just 4% of the trait is explained by direct GWAS effects (compared to a total GWAS heritability of 13% in the same study); and for IQ it’s 14% (compared to a total GWAS heritability of 24% in the same study) [Howe et al. 2022]. Importantly, these are not estimates that will increase over time with more training samples, they are the upper bound on how much “directly” acting variation can be predicted from a GWAS of infinite size.
What is the source of these indirect effects? It is tempting to conclude that they capture genetic variation acting through parenting, and may thus be predictors for the next generation. The field has, in fact, often leapt to this conclusion by labeling such effects “genetic nurture”. However, recent work looking at educational achievement (more-or-less a proxy for IQ from the perspective of genetics) has revealed that these “indirect” correlations may not be acting exclusively within nuclear families (i.e. through parenting), but could be entirely explained by genetic variation in extended family members such as uncles and aunts [Nivard et al. 2024]. Thus, the already weak predictive accuracies described above are expected to be substantially attenuated by environmental factors including — unsurprisingly — broader “dynastic” education and wealth.
The practical implications of these substantial environmental confounds and mediators have already borne fruit (or … whatever is the opposite of bearing fruit). Attempts at genetically-guided “precision education” — where genetics is used to predict student scholastic achievement with the intent of targeting interventions — have essentially been a failure. In real data, much of the predictive power of genetics can be explained by parental education (as shown above), and the little that is left largely disappears when also including the first available school grades, which are themselves much more powerful predictors of future success [Morris et al. 2020] (and perspective from [Janssens 2020]).
Prediction across different contexts is even harder
We have so far not touched on the lack of causality due to correlation of variants, but this has important ramifications for making predictions across different populations. While even geographically diverse populations are genetically very similar (such that if you accounted for all the population differences across continents you would still have 80-90% of the genetic variation left) the correlation across pairs of variants can differ markedly between populations. For predicting within a homogenous population, it does not matter whether one selects the causal variant or a perfectly correlated non-causal proxy, since both will be equally correlated with the trait. But across populations that proxy variant may no longer be correlated with the causal variant, which will lead to bias and inaccuracy in the prediction. This is known as the polygenic score “portability” problem, and it has now been well documented across many studies: polygenic score accuracy decays in rough proportion to the genetic distance from the training population [Ding et al. 2023].
For IQ, there is also the issue of environmental “portability”: because so much of the predictive effect appears to be driven by dynastic environment or stratification, individuals that were raised in substantially different environments may have substantially different dynastic/stratified effects. So what happens to IQ prediction in real data? [Privé et al. 2022] investigated the portability of a large number of polygenic scores trained in White British samples in the UK Biobank. The general lack of population portability was confirmed across many phenotypes: accuracy dropped when predicting into Polish samples, and dropped even further when predicting into Chinese or Nigerian samples (see figure below).
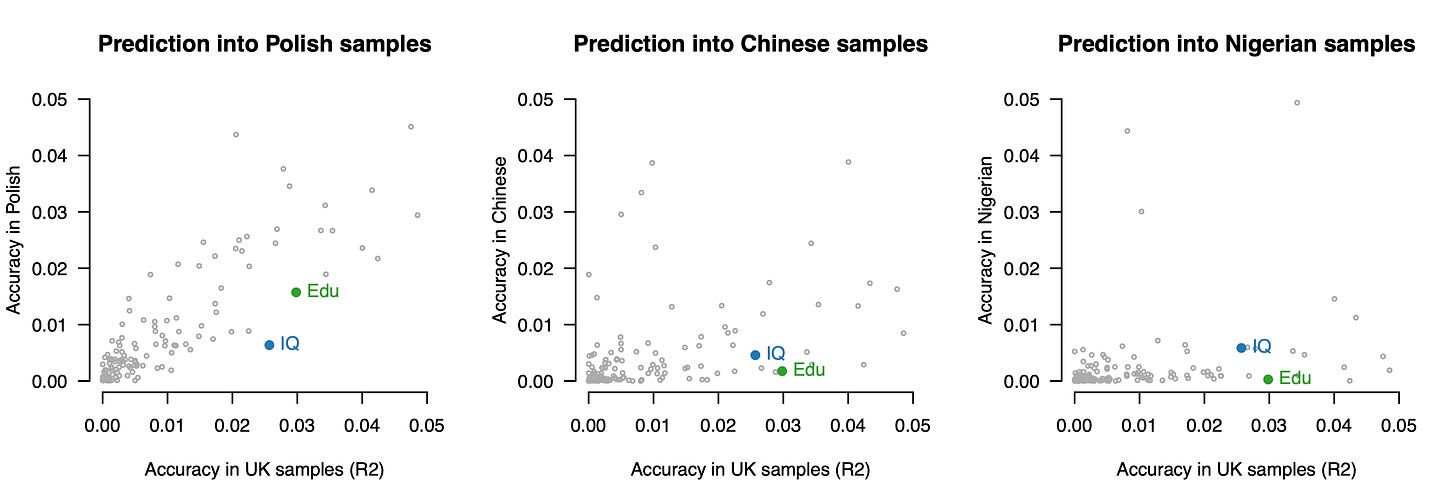
But for IQ specifically the drop in accuracy was unusually large: accuracy in a (held-out) UK sample was 2.6%, compared to 0.6%, 0.5%, and 0.6% for individuals from Poland, China, and Nigeria respectively. The results were similarly sporadic for predicting educational attainment, with an accuracy of 3.0% in the UK sample, compared to 1.6%, 0.2% and ~0% in individuals from Poland, China, and Nigeria. Thus, the already low predictive accuracy can easily drop to nearly nothing, even when predicting across relatively geographically close groups, such as from Western Europe into Eastern Europe. Due to the multiple sources of confounding involved, it is not possible to know in advance how well this predictor will translate into any given population.
Am I just singling out IQ because it challenges my liberal dogmas and slaughters my sacred cows?
Look, I hate getting my sacred cows slaughtered as much as the next person, and I do believe IQ research is often deeply under-theorized. But my critique here is fundamentally methodological: genetic prediction of cognitive phenotypes simply exhibits more confounds than most other traits. As outlined above, the features that primarily drive confounding in genetic studies are (1) stratification (which induces false positives); (2) assortative mating (which induces correlation between causal and non-causal variants); and (3) cultural transmission (which induces correlation between variants causal in one generation and traits in the next). And as it happens, cognitive traits are outliers for all three:
While assortative mating on most traits is modest, it is unusually high for educational attainment (r=0.48) and IQ (r=0.23) [Horwitz et al. 2023].
While the average trait has nearly identical within-family to between-family heritability, meaning it does not appear to be under strong environmental confounding from parental effects, IQ and educational attainment have substantially lower within-family estimates [Howe et al. 2022].
While most traits exhibit “direct” effects that are highly correlated with the effects of non-transmitted/parental variants, the associations with IQ and educational attainment have unusually low correlation, with IQ even showing a negative relationship [Young et al. 2022].
While the heritability of most traits changes very little when adjusting for regional/socioeconomic factors, suggesting that the genetic associations are not strongly confounded by these factors, the heritability of cognitive and socioeconomic traits decreases substantially [Abdellaoui et al. 2022]. GWAS of educational attainment, in particular, exhibits detectable population stratification from very recent (within-UK) structure [Young et al. 2022].
This is all without getting into the thornier questions related to the interpretation of IQ itself: that it changes substantially across generations both in mean and in structure, that it has a markedly different structure at the high end versus the low end, that it correlates strongly with cultural specificity and socioeconomic status, that it has no consistent mapping to brain structure or function, and so on.
But isn’t this all just for fun anyway?
It is worth reiterating the recommendation from the SSGAC I quoted earlier:
… these individual results are not meaningful predictions and should be regarded essentially as entertainment. Failure to make this point clear risks sowing confusion and undermining trust in genetics research. [bold mine]
It’s not that polygenic prediction cannot be entertaining (I guess), but that the distinction between entertainment and information needs to be made very clear. I personally have nothing against tarot cards or palm readers, but if my hospital opened up a tarot-reading wing I would start to get worried about the quality of non-tarot-based clinical care they are providing. The golden era of understanding our own biology means actually providing understanding, not obfuscating between edgy party tricks and serious clinical testing. If Nucleus wants to contribute to this golden era, they have a responsibility to inform their customers about the extremely low accuracy of their scores, as well as the major issues of environmental confounding and population portability. They also need to explain what people should actually do with this low quality information: how does one integrate a highly inaccurate IQ score prediction into their life?2 And for all of the talk about biological understanding and information access, it seems this is an area that Nucleus is very eager to avoid. Here’s how one of the investors explains it on the podcast:
as long as you're not making a clinical assessment, you're just providing the data, but not saying a prescription of you should and should not do these x/y/z clinical things, you are not, sort of, clinically liable
And there you have it: the concern is not to inform, it’s to avoid liability.
One final thought: there does exist an important use-case for genome sequencing and cognitive function, and that is the diagnosis of rare developmental disorders. While polygenic scores for cognitive function have low accuracy, there are very rare single-gene disorders that can lead to severe cognitive or developmental impairment. For such families, a genetic test can mean the end to years of searching for a cause. In fact, this search is so common it even has it’s own deceptively pleasant-sounding name: the “diagnostic odyssey”. Here’s what a diagnostic odyssey can look like: Imagine you have a toddler that starts showing signs of developmental delay; maybe they are unusually non-verbal, or they have extreme difficulty learning to walk, or they’re having seizures, or a bundle of other symptoms. Their physician has never seen anything like it and refers you to a grab-bag of specialists. Each specialist requires a consultation, a series of tests, follow-up visits, following up claims with the insurance company, and so on — all with a child that is struggling to communicate or maybe worsening. At some point you hear about a new company that’s doing all-in-one genetic screening and you look them up. The founders are on a podcast to talk about their product: they fantasize about breeding super babies; they joke with the host about how Neanderthal DNA probably makes you a “fucking idiot”; they periodically and haphazardly come back to the topic of predicting intelligence; when asked about the potential unintended consequences, they make vague claims that “there’s always a balance in nature”; at some point in the interview they suddenly shift tone and talk about the possibility of “eradicating” rare disease through preconception testing coupled with IVF (which they do not offer). Finally, they remind you that, for liability reasons, they are not providing a diagnosis. What’s going on here? Is it a clinical test? Or are we supposed to be entertained? How is your trust in genetics research doing?
Further reading
I’ve written in more detail about related concepts in genetics:
I also recommend the following overview papers:
Coop and Przeworski “Luck, lottery, or legacy? The problem of confounding.”. 2022
Young et al. “Deconstructing the sources of genotype-phenotype associations in humans”. 2019
And the following technical papers:
Veller and Coop, “Interpreting population- and family-based genome-wide association studies in the presence of confounding”. 2024
Morris et al. “Can education be personalised using pupils’ genetic data?”. 2020
Howe et al. “Within-sibship genome-wide association analyses decrease bias in estimates of direct genetic effects”. 2022
“Direct effects” technically refer to variants that, if modified in the individual, would result in a phenotypic change in that individual. It’s worth noting that such changes can still be (and likely are) mediated by society and the environment. The classic example is if red-haired children are forbidden from going to school, then a variant for red hair will have a “direct” effect on educational attainment that is still entirely explained by a cultural mediator. As red hair is highly heritable, educational attainment would also appear to be highly directly heritable in this hypothetical world.
Before I get accused of an isolated demand for rigor — which has become a common rebuttal from those who have none — this is exactly this type of information, including accuracy, portability, and actionability, that was provided in a recent large-scale deployment of polygenic scores for clinical use [Lennon et al. 2024].
One of Sasha's strong suits is that despite being a World leader and authority in human genetics he shows unlimited patience explaining things to people who know absolutely nothing about human genetics, which of course is fine as long as they are willing to learn from an expert.
Please consider enabling TTS. I prefer passively listening as I do other things in general, it's more enjoyable. Thank you
https://support.substack.com/hc/en-us/articles/7265753724692-How-do-I-listen-to-a-Substack-post-
(Request form)
https://airtable.com/shr11c70LRWq9saOb