There were many interesting comments to last week’s post on gene-environment interactions scattered across multiple different social media, which I’ll summarize and (briefly) discuss here. For context, here is the original post:
noted several ambiguities in the terms and definitions used:
I'm confused by the first paragraph which says geneticists usually consider that age and sex fall under E - not sure if that was a mistake, but since it's common practice to adjust for age and sex in ACE models, they wouldn't be part of the decomposition.
Family wealth and inheritance also seem like they would fall under C, not E, but perhaps you meant E as shorthand for anything non-genetic (although people tend to distinguish GxE and GxC).
I also found the definition of interactions slightly confusing & conflating interactions and modifiers [VanderWeele (2009)].
All good points. There’s an important distinction here between language used in GWAS and language used in twin models. In GWAS, the study population is typically unrelated so there is no “shared environment” as such, and all environmental terms are lumped in as E. In twin models, the study population consists of pairs of siblings (with different zygosity) so the environment can be decomposed into the shared (C) and non-shared (E) variance component. Whether C captures “objective” shared environments like wealth (i.e. measurable environments in both siblings) or “effective” shared environments (i.e. those that make siblings more similar) is a matter of debate (see [Turkheimer & Waldron (2000)]. Both GWAS and twin models also typically adjust for Age and Sex as covariates, but note that this does not adjust for GxAge or GxSex interactions that are uncorrelated with the marginal terms.
The referenced paper distinguishing interactions from effect modifiers is also worth reading. The author uses a causal framework to define interactions as “the effect of 2 exposures together to be different from the combination of the 2 effects considered separately” and modification as (paraphrasing) the effect of the primary exposure varying across subpopulations defined by some other variable. They key distinction here is that, for an interaction, both G and E are necessarily causal on the outcome, whereas for effect modification E can just be non-causally correlated with some other unmeasured cause. I’ve repurposed the key figures from the paper in the context of GxE below:
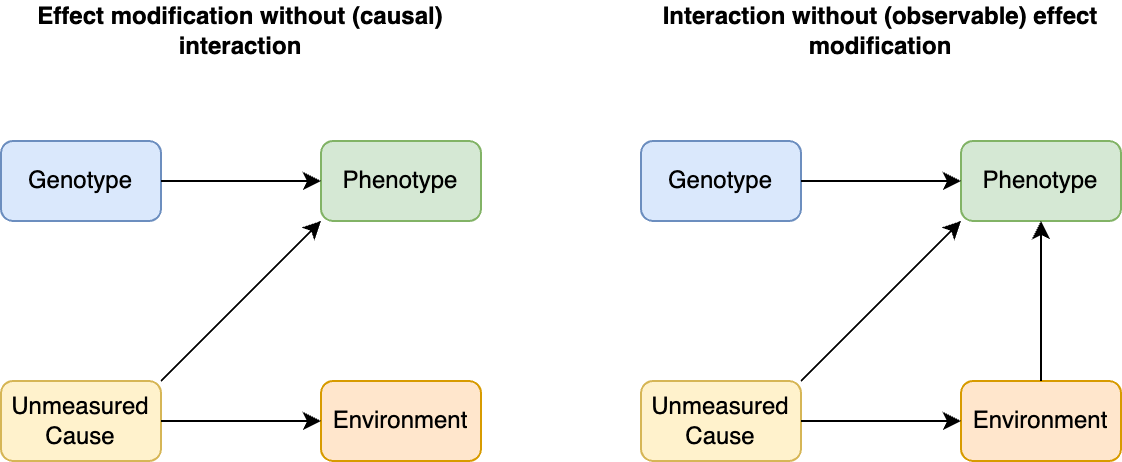
On the left, E does not have a causal effect on the phenotype and is therefore an effect modifier. This is important because intervention on E would not change the effect of the genotype. On the right, E does have a causal effect on the phenotype that interacts with the genotype. However, the author points out that if E and the unmeasured cause have compensatory effects, then this interaction may be cancelled out in the observational data. Thus an observed effect modifier is neither necessary nor sufficient to have an underlying causal interaction. All of the examples I described in the post were observational (in fact, since the cited studies mostly used population rather than within-family analyses, even the G→E path may not be causal), so it would be more accurate to describe them as GxE effect modifiers. This causal distinction is important for understanding how we can intervene on environments to change outcomes. And on the topic of causal interpretations …
George Davey Smith (mendel_random) noted that GxE interactions can also be leveraged to improve causal inference with Mendelian Randomization:
Some interactions are robust, and can be used powerfully in causal inference. These include interactions with zero relevance point / negative control populations, demonstrating consequences of alcohol intake on health eg [Chen (2008)] and [Cho (2015)] and a general formulation for using interactions for effect estimation in Mendelian randomization [Spiller (2022)] and [Spiller (2019)].
This is a great point that I had not considered at all in the original post: GxE can be leveraged to obtain more precise estimates of the causal effect of various E’s on each other. Mendelian Randomization (MR) is typically used to infer the effect of an exposure on an outcome using genetics as an “instrument”. But this approach is very sensitive to pleiotropy: when the genetic instrument is causal for both the exposure and the outcome. The motivation in using GxE is that it gives you a source of heterogeneity that can be exploited to test and/or correct for pleiotropy. If you can find (or extrapolate to) an environment in which your instrument does not influence the exposure (the so-called “no-relevance group”), and it still effects the outcome, then you know you have a pleiotropy problem and can try to correct for it. The GxE itself is not the parameter of interest, but it becomes a tool to better evaluate E→trait effects. It is a very clever approach. And again on the topic of causal interpretations …
Nilanjan Chatterjee noted several challenges for interpreting GxE from biobanks:
Great summary. A few thoughts
(1) For time varying factors like the BMI and smoking example, can we really study G by E interaction with cross-sectional analysis when we don’t know what comes first.
(2) For studying disease risk similarly I don’t know how to interpret any G by E finding for time varying exposure unless incidence disease outcome is being used. I insist this point as I m seeing reports of PRS by context interaction in studies based on EHR where complete incidence outcomes cannot often be clearly defined. In UKB, where incidence disease outcomes can be clearly defined, there is a very little evidence of non-multiplicative effects of PRS and E.
(3) What is the impact of population stratification that can create G-E correlation and also confounding through other mechanism for G-E interaction study.
These are important points to keep in mind. Reverse causality is a particular concern in cases where environments can actually be consequences of the outcome. I think that quasi-experimental methods (e.g. using existing policy interventions in conjunction with genetic data) can help resolve some of the issues around time-varying or bi-directional effects. I am also optimistic that family-based analyses of direct and non-transmitted genetic variants might provide “two bites at the apple” by distinguishing early parental influences from later direct influences on some outcome. See the recent review by Benjamin et al. (2024) for more examples. And on the topic of bidirectional and time-dependent effects …
Greg Kohn noted parallels to interactions in animal behavior:
The discovery of unexpected GxE effects has been a staple of a small group of researchers working in the development of animal behavior. It’s hard to study though because, well, it’s hard to uncover non-obvious unexpected things on first principles [Turvey et al. (2017) “Non-obvious influences on perception-action abilities”].
This paper builds off from a very interesting experimental observation in rats (“Rats fed regular chow related to their surroundings by means of geometry. Rats fed an energy-rich diet did not; they related to their surroundings by means of features (luminance and pattern).”). The authors cite multiple other examples of development in the animal world where environmental stimuli lead to completely unexpected behaviors. In other words (as the title suggests) environmental influences can be non-obvious. They conclude that “in respect to development and learning, all experiences in their respective time scales might be expected to contribute—the logically major and minor, the obvious and the non-obvious, the prolonged and the instantaneous, the recurring and the once only”. This very much echoes my sense that, at least for some traits, the patterns of GxE may be so idiosyncratic as to be causally intractable.
More hereditarians need to take articles like these seriously imo. I’ve seen lots of sneering but no attempts at a constructive “rebuttal” (not that this should be a goal but you understand) and this is coming from someone who is biased against an env model.
What do you think of this?
"The 'genome-wide complex trait analysis (GCTA)' method (Yang, Lee, Goddard, Visscher) has appeared to partially resolve the 'missing heritability' problem: that the sum of GWA-identified SNPs explain only a small fraction of heritability. It estimates the variance explained by a constellation of common SNPs from the whole genome for a complex trait, rather than testing the association of any particular SNP to the trait. Using the PGC sample, it was estimated that SNPs account for 23% and 25% of variation in liability to SZ (Lee et al., 2012b) and BD (Cross-Disorder Group of the Psychiatric Genomics, 2013), respectively. They also estimate that 1) this is mainly due to common causal alleles, 2) they must be evenly spread across chromosomes since the variance explained by each chromosome is linearly related to its length, 3) the genetic basis of SZ is the same in males and females and 4) as expected, a disproportionate amount of variation in liability is attributable to a set of 2725 genes expressed in the CNS. Furthermore, using only unrelated subjects and the same SNP genotypes, a 68% genetic correlation between these disorders was found. Although most of the SNPs responsible for the variance explained are not yet identified, the rationale is that they will be, as GWAS sample sizes increase and more accurate estimation of the effect size of each SNP is achieved."
Prata, D. P., Costa-Neves, B., Cosme, G., & Vassos, E. (2019). Unravelling the genetic basis of schizophrenia and bipolar disorder with GWAS: A systematic review. Journal of psychiatric research, 114, 178–207. https://doi.org/10.1016/j.jpsychires.2019.04.007