Gene-environment interactions: ubiquitous yet undetectable
Polygenic models have revealed widespread GxE hiding under the surface of trait heritability
Identifying interactions between genetic variation and the environment (GxE) has been a great white whale in human genetics: commonly observed in other organisms but nearly impossible to detect for individual variants in humans. Several recent papers have instead sought to quantify the total genome-wide contribution of GxE interactions, as well as the biological models they are compatible with. What has emerged is a blanket of subtle, highly polygenic effects across many contexts.
How do genetic variants interact with the environment?
Let’s start with some definitions. Geneticists tend to be self-centered and refer to the “environment” (E) as any process that is not the direct effect of genetic variant: conventional exposures like pollution are considered “environment”, but so are behaviors like smoking, family influences like parenting or inherited wealth, even endogenous processes like age and sex. Next, a statistical interaction occurs when two influences together have a different impact than just the sum of their parts. A genetic variant increases the trait by +2 points and an environmental factor increases it by +3 points, but carrying the variant in the environment increases their trait +50 points (or zero points) — that’s an interaction1. Interactions can be interpreted in two ways: the effect of the variant on the trait changes in different environments (the variant is moderated by the environment); or the effect of the environment on the trait changes for variant carriers versus non-carriers (the environment is moderated by the variant). Similar intuition applies to continuous environments.
Most common traits are highly polygenic, so we want to think about GxE in the context of many genetics influences. Several such models have been proposed:
In the classic or locus-specific model, individual variants interact with the environment in distinct ways. Imagine that alcohol consumption induces many different biochemical changes, leading to an increase in the influence of some genetic variants on BMI and a decrease in the influence of others [Young (2016)]. For each variant, the effect size estimated in drinkers versus non-drinkers will show idiosyncratic differences. For the same trait measured in two environments, this will manifest as an imperfect genetic correlation.
In a uniform amplification model, the entire genetic component of a phenotype behaves differently in one environment versus another [Mostafavi, Harpak et al. (2020)]. Imagine that in low quality schools, kids with genetic variants for poor eyesight get ignored/left behind by their teachers. Over time, these small genetic differences are amplified by the environment and the heritability of educational attainment grows. In high quality schools, kids with the same eyesight mutations receive free eye exams and glasses and do just fine in school: here, the influence of genetics on educational attainment is instead moderated by the environment. Since the environment is acting on eyesight, the effect of all eyesight variants will then differ uniformly across the two environments. This is sometimes referred to as a “gene-environment transaction” because genetic and environmental influences trade off in different contexts. For the same trait measured in two environments (e.g. in low/high quality schools), this will manifest as a perfect genetic correlation but a significant difference in heritability.
In a proportional amplification model, uniform amplification is happening on genetic factors and on environmental factors [Zhu et al. (2023)]. Both the genetic and environmental variance of the phenotype grows proportionally. Imagine genetic and environmental factors influence a metabolic pathway that then influences BMI, but the pathway remains active for longer in men than in women. In this case, both the magnitude of the genetic influences and the environmental influences on the pathway increase in men. Sex is acting as a proportional amplifier on both inputs. For the same trait measured in two environments, this will manifest as a perfect genetic correlation with no difference in heritability but a difference in the overall phenotypic variance (e.g. higher BMI variance in men).
We can visualize these models in the toy example below: for locus-specific GxE, the genetic effect-sizes vary sporadically between environments; whereas for amplification they are all increased in magnitude to a similar extent.
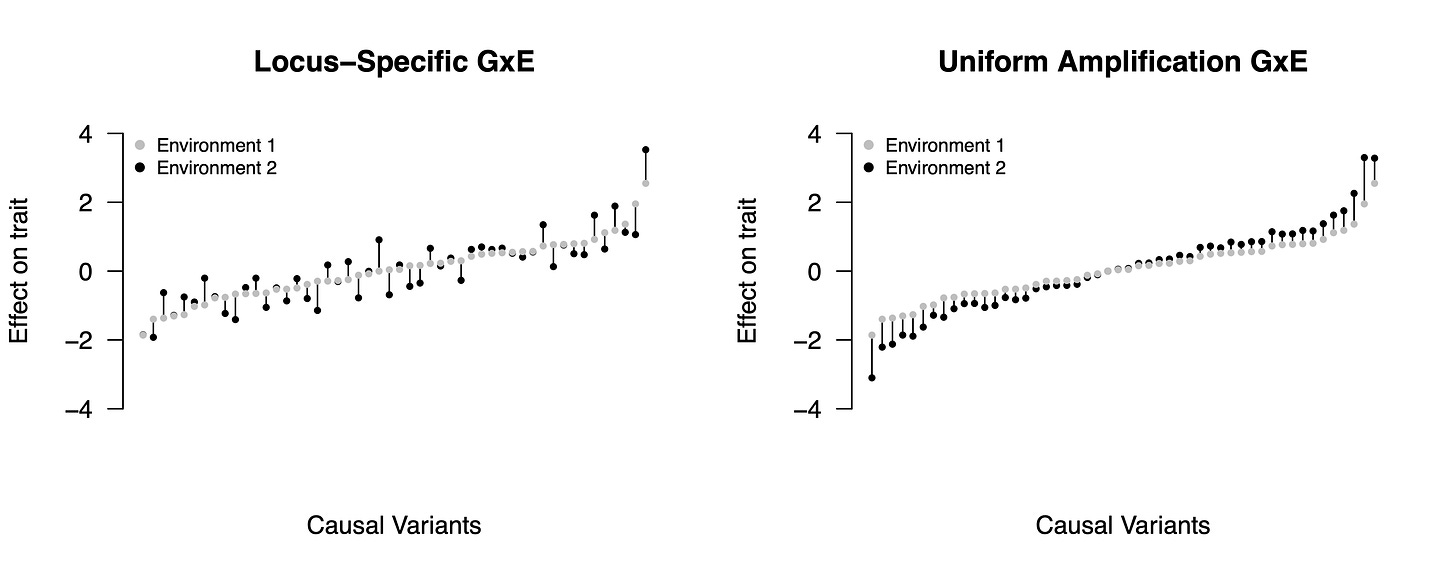
The above is a set of largely statistical explanations, but there is also a conceptual perspective: the further an environmental modifier is from the genetic influence, the more it will amplify both genetic and environmental effects and look like the proportional amplification model ([Zhu et al. (2023)], [Durvasula et al. (2024)]). An environmental modifier of the FTO gene is very “close” to the genetics, and thus locus-specific. An environmental modifier of a highly heritable trait like eyesight is further upstream of many variants but still mostly impacting a genetic pathway, so leads to uniform amplification. An environmental modifier of a less heritable / more diffuse pathway like metabolism is upstream of both genes and environment, and so leads to proportional amplification.
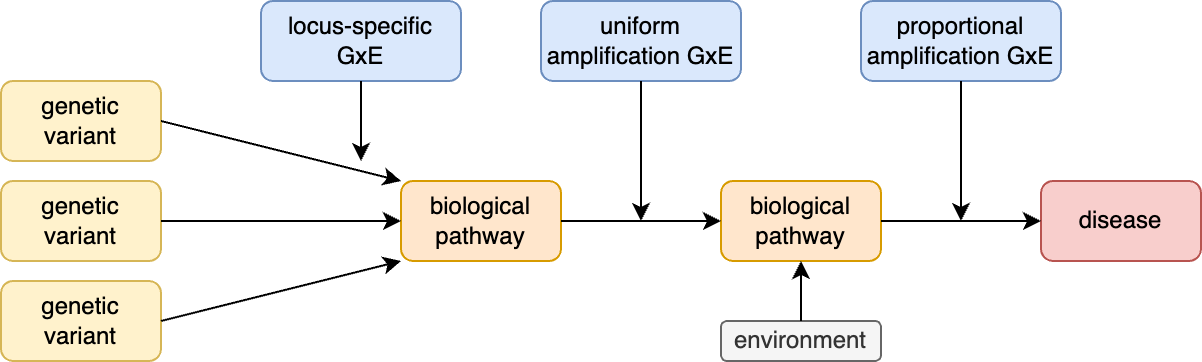
Polygenic GxE is widespread
The typical approach for quantifying polygenic GxE is to collect genetic and phenotypic data from a very large number of individuals in multiple environments. Then add a GxE interaction variance/heritability component to standard estimators (which do not attempt to distinguish the models)2 or estimate the different model parameters described above. The obvious limitation is that the putative environments actually need to be known and measured, which can be a challenge when (as is now common) using clinical biobanks that do not collect social/epidemiological surveys.
Proof of principle: BMI
In an early study of polygenic GxE, [Robinson et al. (2017)] used the variance component approach to estimate the GxE heritability of BMI with various lifestyle factors. Their focus on BMI was motivated by the large observed gaps in heritability estimates from twins (which can be inflated by GxE interactions; more on this later) relative to other relationship classes3 .
They start with a ubiquitous “environment”: age in a cross-sectional cohort. Age is an interesting biological variable because it can capture multifactorial environmental influences, including both external exposures (psychological stress) and endogenous biological factors (DNA repair) that change/accumulate over time. The authors observe a highly significant SNP-age interaction for BMI, with a substantial GxAge heritability of 8% on top of a standard additive heritability of 21%. This interaction was most consistent with the locus-specific model, as genetic correlation was significantly low between young and old individuals (rg = 0.56 s.e. 0.19) whereas total heritability did not change substantially. Thus, while genetic variation appears to contribute the same magnitude over the life course, the underlying mechanisms are changing in idiosyncratic ways. As a negative control, no such differences were observed for height. Perhaps age modifies the function of many very basic biological processes.
Next, BMI was adjusted for age and sex and evaluated for GxE heritability with eight (self-reported) lifestyle variables. A model with a GxE term provided a better fit for every E variable, supporting the general hypothesis that many environmental interactions are involved. An individually significant interaction was also observed for smoking, which explained 4% (s.e. 0.8%) additional variance in BMI. Summing up across all eight factors explained 7.5% additional variance compared to a marginal heritability of 22%. Environmental interactions could thus increase the explained by variance by ~1.4x.
Many trait-environment pairs
The variance component approach has since been extended with efficient statistical methods that can analyze a large number of individual environmental factors and traits. [Di Scipio et al. (2023)] developed and applied a fast variance component method to multiple biomarker measurements in the UK Biobank and the environments of Waist-Hip Ratio (WHR), physical activity, and smoking. In total 15/39 trait-environment pairs tested exhibited significant GxE heritability. In some cases, these terms were quite substantial: triglyceride levels, for example, having an additive heritability of 21% and GxE interaction heritability of 7% with WHR. A similar method4 was developed by [Pazokitoroudi et al. (2024)] and applied to 50 quantitative traits in 4 environments (i.e. 200 pairs) of which 68 pairs were found to exhibit significant GxE heritability. For example, 21/50 traits showed a significant interaction with smoking, with an average GxE heritability of 6% of the additive heritability. Thus, individual trait-environment interactions, while small on average, appear to be pervasive. But what about simultaneous interactions with many environments?
Multivariate environments
[Kerin et al. (2020)] proposed a multivariate model that learns how to combine many environmental measures into a linear “environmental score” (a very interesting method that has somehow mostly flown under the radar). Similar to a polygenic risk score, this environmental score is a weighted sum of all included environments, which aims to maximize the corresponding GxE effects. The total multi-environment GxE heritability can then be estimated by treating the learned score as a single fixed environmental variable. The method was applied to BMI and three blood pressure related traits together with 42 behavior/lifestyle environmental variables (yet again in the UK Biobank). For BMI, multivariate GxE heritability accounted for 6-14% of the trait variance (depending on the scaling). Significant GxE heritability was also observed for pulse pressure, explaining 13% (s.e. 3%) of the trait variance compared to 23% (s.e. 5%) for additive genetics. Interestingly, this GxE heritability was largely concentrated in low frequency variants, to a much larger extent than the additive component, suggesting potentially unusual evolutionary dynamics5. The results from all studies are summarized below. Aggregating environments into a composite environmental score further increased the proportion of trait variance that could be explained beyond single environment interactions.
Amplification GxE is widespread
While the above studies sought to quantify the total amount of trait variance that could be explained by interactions, other work has evaluated how consistent the patterns of GxE are with the different conceptual models.
Support for models of GxE across traits
[Mostafavi, Harpak et al. (2020)] quantified heritability and genetic correlations for three very different traits: BMI, blood pressure, and educational attainment. For all three traits, significant differences in heritability were observed across different environments: BMI and age, blood pressure and sex, educational attainment and socioeconomic status. The differences for educational attainment were particularly striking, with a heritability of ~11% for the highest SES group versus ~21% for the lowest. In other words, different environments could amplify (or dampen) the total association of genetic variation with educational attainment by nearly 2-fold. Importantly, the differences in heritability could not be explained by lower environmental variance, and were consistent with proportional amplification.
[Durvasula et al. (2024)] expanded upon this approach and analyzed 33 complex traits in 10 environments. They found pervasive support for GxE from all three biological models described above: 19 trait-E pairs consistent with locus-specific GxE; 28 pairs consistent with amplification; and 15 pairs consistent with proportional amplification. Though each instance of GxE explained only 0.6% of the trait variance on average (for continuous traits, the results for binary traits were more complicated due to scaling), 27/33 traits showed significant evidence for at least one form of GxE, with amplification accounting for the majority of assigned models.
Support for models of GxE mixtures within traits
Rather than assume that each trait-environment pair belongs to a specific GxE category, [Zhu et al. (2023)] proposed to quantify the mixture of GxE models acting on the trait. Using GxSex interactions as a pilot, they estimated the fraction of genetic variance that was consistent with higher amplification in males, or in females, or equal (in addition to departures from perfect genetic correlation for each class). Amplification was widespread, and for 13/27 traits, the majority of non-zero variants were estimated to have larger magnitude in one of the sexes: meaning most variants were involved in amplification in the same direction. However, the mixture proportions varied across traits: at one end, arm fat-free mass had 92% of the non-zero effects larger in males and just 3% larger in females; at the other end, height had 29% of the non-zero effects larger in males, 9% larger in females, and 62% equal between sexes. The schematic at the start of this post is thus an oversimplification; in practice, GxE can be a mixture of amplification and dampening with a general tendency towards one or the other.
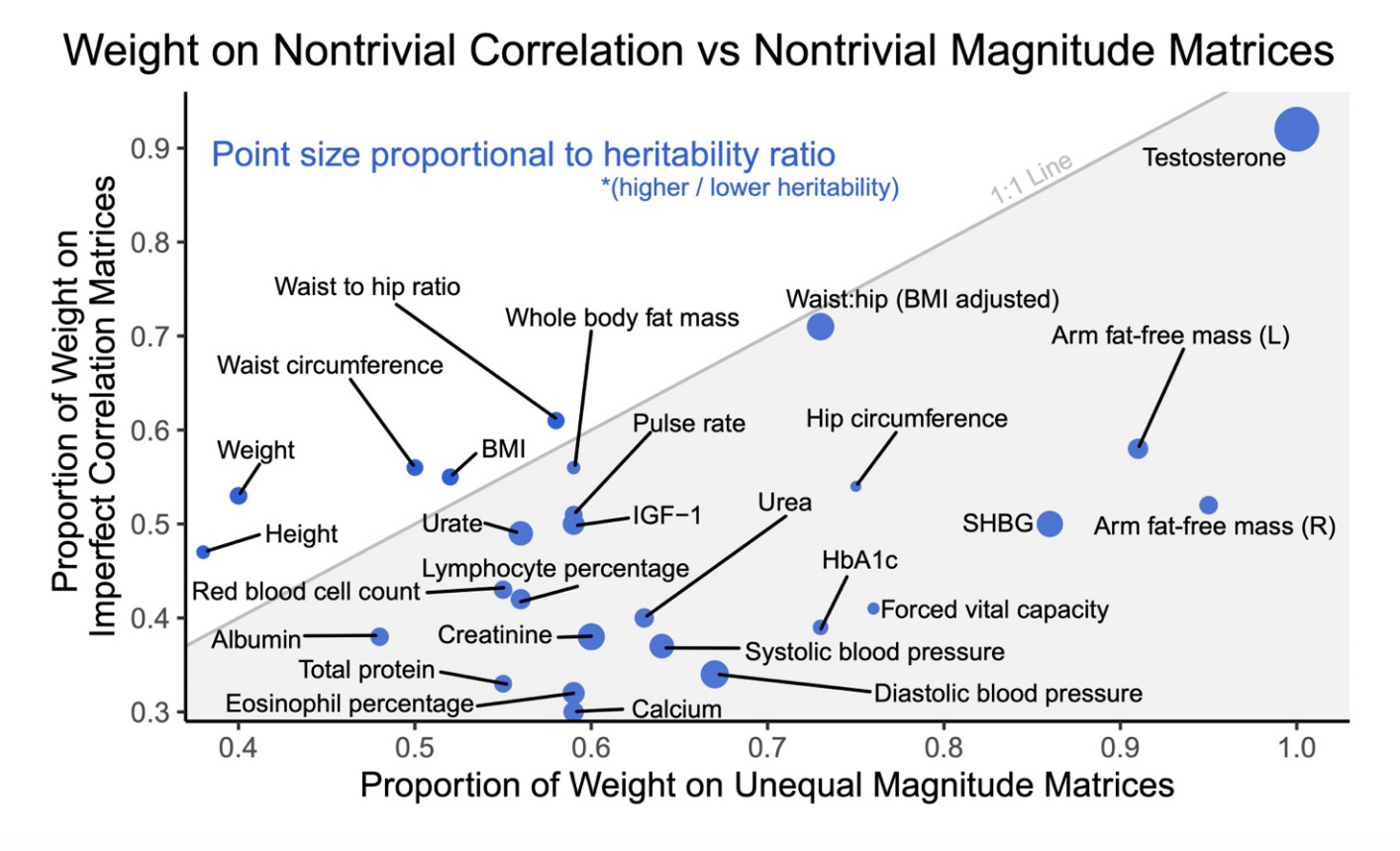
[Nagpal et al. (2024)] expanded this mixture analysis to seven common diseases in the context of pairs of 75 different exposures (i.e. 75 choose 2 = 2,775 exposure pairs in total). Amplification was again pervasive, but more interestingly, genetic effects were systematically amplified in the more high-risk/adverse exposure combinations. For example, genetic effects on Coronary Artery Disease were 2x higher in smokers with low omega-six fatty acids (the good fatty acids) compared to non-smokers with high omega-six fatty acids. Across all seven traits, a significant positive correlation was observed between having more variants amplified in the higher risk exposures and having more GxE. Thus, the previous example of educational attainment exhibiting lower heritability in high SES environments is consistent with a broader pattern where high SES / low risk exposures interact with and dampen the influence of harmful genetic variation (though that these are still just correlations and there can be multiple causal explanations).
These trait-specific patterns of amplification mixture are intriguing in and of themselves. Why do some traits have much more uniform amplification than others? What regions of the genome tend to be under consistent or dynamic amplification across traits and why? We clearly have a lot more to learn.
Homogamy is also an environment
I mentioned various examples of an environment above but I think one under-appreciated source of environmental influence is cultural structure: specifically, the extent and structure of homogamy (aka assortative mating). Phenotypic assortative mating makes spouses and siblings more genetically similar than they would be, increases the correlation between otherwise uncorrelated variants, and, as a consequence, increases the overall genetic variance in the population (see prior derivations and examples). That means a sub-population that is undergoing assortative mating will have higher heritability than a sub-population that is mating randomly. From the models above, this will look like amplification GxE, even though the “E” here is just mating patterns. In addition to the real increase in genetic variance, estimators of heritability also tend to be inflated by assortative mating [Border et al. (2022)] which will lead to even more estimated amplification.
This is all getting quite abstract, so let’s run some simulations to see what it would look like with realistic parameters. We’ll generate a phenotype that consists of 30% genetic variation, 10% direct cultural transmission (i.e. influenced by the mean parental phenotype) and the rest random environment. Then we split the population into two and have one mate randomly while the other undergoes assortative mating with spousal correlations of 0.40 (similar to what has been observed for educational attainment). To reiterate: the environment in each sub-population is exactly the same, all we’ve changed is how spouses are selected. Finally, we estimate heritability within each sub-population and in the combined population using a standard method (Haseman-Elston regression).
Several points stand out. First, the “true” heritability is higher than the 30% “direct” heritability we induced in all groups: this is because population-level heritability measurements include some of the effects of parental phenotypes that become correlated with genetics (it is only within-family estimates that would give us the true 30% direct effects). Second, the true heritability is even higher in the population with assortative mating: this is because assortative mating amplifies the influence of cultural transmission, effectively making the phenotype appear more (non-causally) heritable. Third, the estimated heritability is further biased upwards in the populations undergoing assortative mating: this recapitulates the findings in Border et al. (2022) about bias in estimation. Finally, because assortative mating increases the genetic variance (and we have held the environment constant), the total phenotypic variance also goes up. These two populations thus appear to be undergoing amplification GxE entirely explained by differences in homogamy.
Twin estimates of G are inflated by GxE
All of the studies discussed so far used population-level molecular estimators of heritability in homogenous, unrelated individuals. But as long as molecular genetics continues to cite twin study estimates as a heritability reference point it’s worth checking in on how twin studies are impacted by GxE. The Classical Twin Design ACE model assumes no interactions at all. Worse, because interactions between genetic variation and the shared/family environment are fully shared by MZ twins and 1/2 shared by DZ twins, their contribution will be entirely assigned to the additive heritability component. Entirely? Yes, entirely. Every single genes-by-shared-environment interaction will be counted as additive genetics. Each of the GxEs observed above (and all the others that are yet to be identified), if they also operate through the environment shared by siblings, will get counted as genetics. Every single one. [Purcell (2002)] has the derivations, but let’s again confirm with simulations.
We start with a phenotype that is composed of 20% additive genetics and 10% shared environment and we progressively add more interacting environments, with each interaction explaining 5% of the trait. Generate twins and run the ACE model to get “twin heritability”. Then take one individual from each family and estimate “population heritability” by simply computing the squared correlation between their genetic value and the phenotype. As expected, as each new GxE interaction is added its contribution is counted as additive heritability by the twin model, while the population model is not impacted.
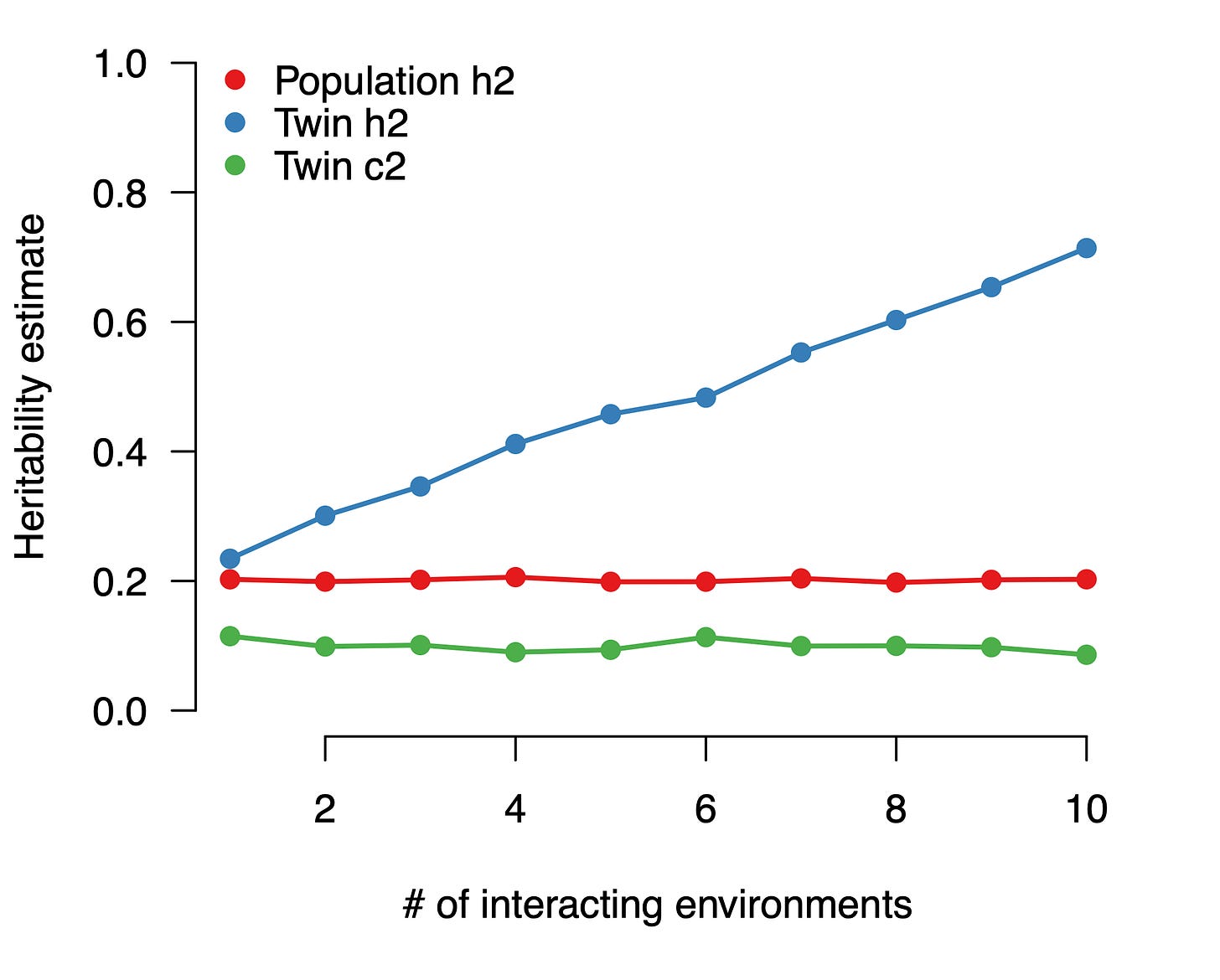
The gap between low molecular heritability estimates and high twin heritability estimates could thus be explained by (a) rare and other genetic variants missed by the former (“missing heritability”); or (b) GxC interactions incorrectly assigned to genetics by the latter (“missing environments”). Could it be that twin studies have been estimating gene-environment interactions this whole time?
Takeaways
So what did we learn? On the one hand, there were few instances of substantial locus-specific GxE, most notably for BMI (with age and smoking) [Robinson et al. (2017)] and for testosterone (with sex) [Zhu et al. (2023)]. On the other hand, amplification GxE appeared to be widespread — present for nearly all traits and many environments — but with each individual environment explaining only a small amount of the trait variance on average [Di Scipio et al. (2023), Pazokitoroudi et al. (2024), Durvasula et al. (2024)]. Across traits and exposures, genetic variation tends to be amplified in more hazardous environments [Nagpal et al. (2024)]. When aggregating multiple environments into “environmental scores”, the total interaction effect can also accumulate substantially, though this approach has not been widely applied [Kerin et al. (2020)].
Why have individual interacting variants been so difficult to detect?
Now that we have a sense of the trait variance explained by individual interactions, the reason why GxE has been a white whale becomes obvious: statistical power. If we optimistically assume a trait with 1,000 causal variants (on the lower end for common traits), a main effect h2 of 20%, and a GxE h2 of 2% (at the higher end of what has been observed on average), one needs ~500k individuals to achieve >50% power for the main effect but 5M individuals to achieve >50% power for the interaction effect (both at genome-wide significance). With an additional multiple testing penalty for each environment that is considered, many millions of individuals will likely be needed to identify a sizable fraction of GxE interactions, if they can be identified at all.
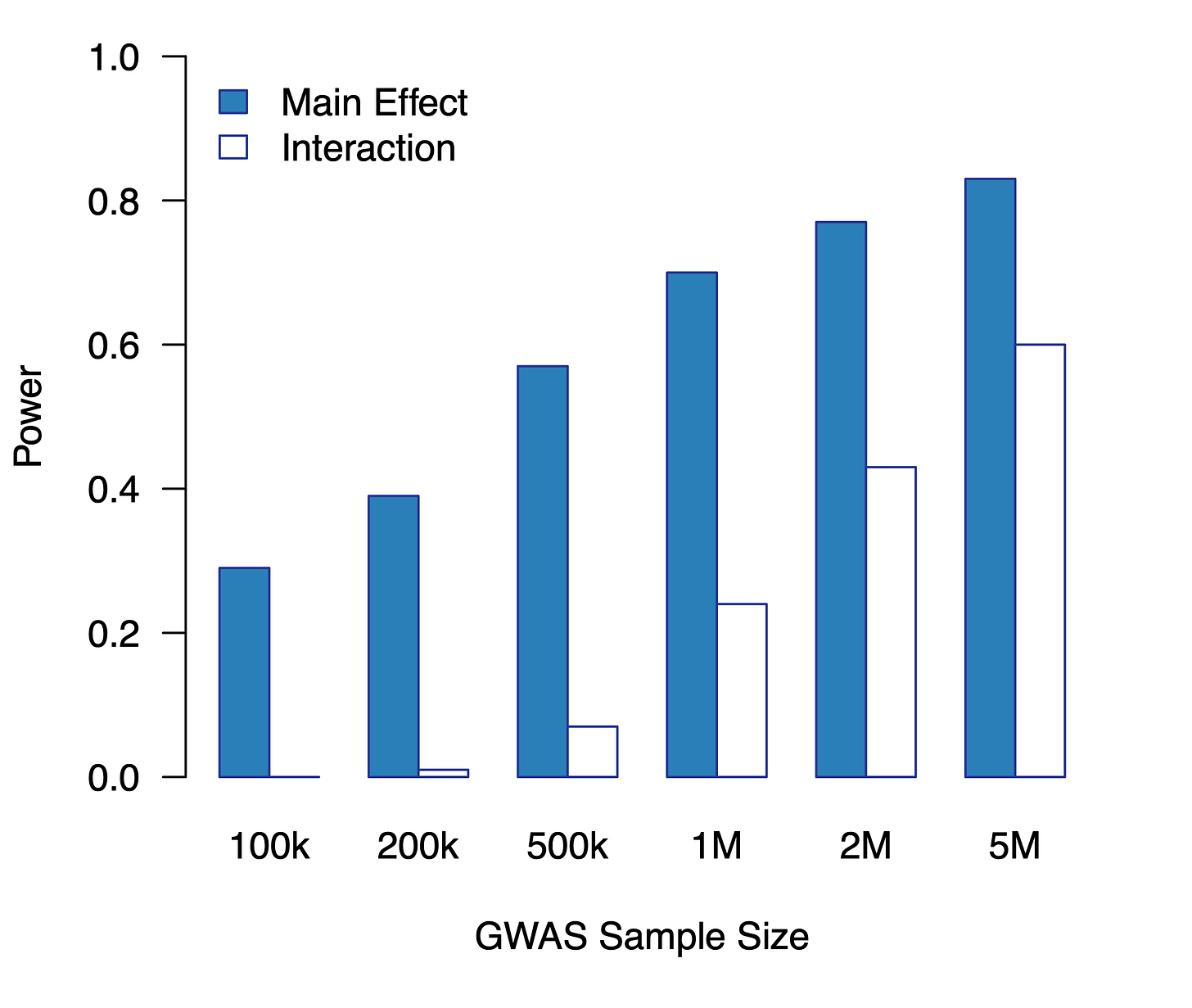
What about environmental factors or “indices”?
One can imagine aggregating environments into low dimensional factors that individually explain more of the environmental variance and reduce the testing burden (similar to the “environmental score” model in Kerin et al.). How much can we reduce the dimensionality of the “exposeome”? A recent study [Carey et al. (2024)] conducted a factor analysis in the UK Biobank using a large number of measurements including health records, lifestyle factors, sociodemographics, environmental measurements, etc. The analyses settled on 35 factors (7% of the items used) explaining ~22% of the variance across all items. Using 7% of the data to explain ~22% of the variance is certainly not nothing, but it suggests the space of environments is quite high-dimensional.
Interpretation of heritability
The interpretation of GxE heritability does not fit neatly into the typical nature/nurture buckets. This is especially true for amplification GxE under high-dimensional environments: at any point in time each of us resides at some location in a complex environmental landscape that is simultaneously amplifying or dampening the influence of thousands of genetic variants. Environments defined by behaviors or biological processes are themselves weakly heritable, further bringing genetics into entanglement.
There are also important implications for genetic prediction, since prediction is intended to be prospective but environments are only known retrospectively. For largely fixed or deterministic environments like sex or age, one can train environment-specific predictive models (and Zhu et al. demonstrate that this can be effective for sex as an example). But for socioeconomic status or adult Waist-Hip Ratio or multivariate environmental composites one would first need to accurately predict the non-genetic future, and then propagate through the interaction with genetics. What ostensibly makes genetic predictors special is that they are fixed at conception; if we instead take the product of a fixed quantity (G) and a variable one (E), the result is no longer fixed and we are left with something that behaves just like any other epidemiological risk factor. If the missing heritability gap is indeed explained by stochastic GxE, then genetic prediction may never reach the heritability estimated by twin studies. At best, a genetic predictor may be able to tell you whether your prediction is more or less uncertain due to your position in the environmental landscape (e.g. [Hou et al. (2024)]).
Implications for evolutionary models
An interesting and largely unexplored implication of GxE is for our understanding of human evolution. The finding in [Kerin et al. (2020)] that GxE heritability was greatly enriched in low frequency variants is provocative6, but was only observed for a handful of traits and has not yet been recapitulated in subsequent work [Pazokitoroudi et al. (2024)]. We can additionally think of modern-day environmental measurements as murky windows into the past: the influence of genetics in a low SES environment today may be a crude proxy for the influence of genetics in a typical environment of, say, the 1970’s. There have even been calls to quantify GxE in remote human populations that are still undergoing “ancestral” environments as a proxy for historical human development.
From this perspective, lack of substantial locus-specific GxE may be consistent with individual genetic mechanisms being largely non-adaptive: there are simply very few genetic variants that decrease (or do nothing to) a trait in one environment and then go on to increase it in another. Rather, changes in environment would amplify/dampen the overall magnitude of genetic effects on fitness (via fitness related traits), which in turn would increase/decrease the strength of polygenic adaptation across most variants. It has also been argued that heritability increases with better environments / higher quality of life — implying a potential acceleration of selection over the course of human development — but the GxE data suggests the opposite: in environments that are less harsh, the influence of genes is dissipated and selection would therefore be weakened.
Further Reading
There have been several interesting reviews of GxE touching on different aspects:
Herrera-Luis et al. (2024) Gene–environment interactions in human health. Focusing on statistical methods.
Boye et al. (2024) Genotype × environment interactions in gene regulation and complex traits. Focusing on analyses of molecular data.
Gibson and Lacek (2020) Canalization and Robustness in Human Genetics and Disease. Focusing on the evolutionary context.
Update: I discuss some comments/feedback in response to this article in a brief follow-up:
Keep in mind that statistical interactions are not the same as biological interactions: two influences can have a complicated biological relationship within an individual but still have an additive impact on average in the population. Interactions also depend on the scale that the phenotype was measured in, an interaction on height can look like an additive effect on the log of height and vice versa. Without knowing the biological mechanisms one cannot know the “right” scale, though we tend to expect parsimony and are disappointed if a simple scale transformation can make all/many interactions disappear.
Some intuition on these models. For variance component estimators (e.g. GREML) the standard model is quantifying whether individuals who are genetically similar are also phenotypically similar; then the GxE term quantifies whether those individuals who are genetically similar and in the same environment (e.g. smokers) are more phenotypically similar than those that are genetically similar but in different environments. For variant-level estimators (e.g. LDSC), the standard model is akin to the sum of squared causal genetic effects on the trait in one environment; then the GxE model is akin to the sum of the squared causal effect size differences between the environments. GxE components can also be estimated using polygenic scores but this parameter has a more complicated interpretation due to the reliance on a training sample.
“Taken together, these results support a h2 for BMI of ~0.4 (refs. 6–12) and a systematic inflation of BMI heritability estimates in classical twin studies. We now explore whether genotype–age and genotype– environment effects could contribute to this inflation or whether it is due simply to confounding between relatedness and the degree of shared developmental environment.” ~ Robinson et al. (2016)
I’m glossing over the details but there are actually important and interesting differences in how the methods handle heterogeneity of noise between environments.
“In contrast, we found that variance explained by GxE effects was overwhelmingly attributed to low-frequency SNPs (MAF <0.01), especially those with low LD. However, we are not aware of any evolutionary theory that has been extended to model the MAF distribution of GxE effects.” ~ Kerin et al. (2020)
In very broad strokes, the more a trait is driven by rare/low-frequency variants, the more we tend to think of it is having been under selection at some point in the past. GxE effects being driven by lower frequency variants could thus imply a history of stronger selection specifically on the GxE component (though no such formal theory has been developed for GxE; see previous footnote).
I need time to absorb this.
Great summary. A few thoughts
(1) For time varying factors like the BMI and smoking example, can we really study G by E interaction with cross-sectional analysis when we don’t know what comes first.
(2) For studying disease risk similarly I don’t know how to interpret any G by E finding for time varying exposure unless incidence disease outcome is being used. I insist this point as I m seeing reports of PRS by context interaction in studies based on EHR where complete incidence outcomes cannot often be clearly defined. In UKB, where incidence disease outcomes can be clearly defined, there is a very little evidence of non-multiplicative effects of PRS and E.
(3)what is the impact of population stratification that can create G-E correlation and also confounding through other mechanism for G-E interaction study.
Nilanjan