Last week’s post on the fundamental genetic differences between IQ and height brought in a number of questions and comments, some interesting and some frustrating. Much of the discussion happened at Hacker News and the Slate Star Codex subreddit1, where two broad points stood out. First, some commenters got really hung up on their priors from classic twin/family estimates, even though the post was entirely about the evidence from “modern DNA science” (as described in the original Atlantic article) including parameters like direct/indirect effects that twin studies cannot estimate. Most of this was reasonable confusion (“why is Howe et al. talking about heritability of 24% when I’m used to much higher estimates”) and simply highlights the relatively poor job geneticists have done at explaining how our work fits into historical quantitative genetics. Second, it became clear that work within molecular genetics had also evolved very quickly, such that a lot of the literature is woefully out of date: papers from 2017 and earlier contain outright erroneous claims (completely ignoring indirect effects), papers from 2018 make predictions that have already been proven wrong (grossly underestimating the bias due to indirect effects), and even papers from early 2024 use terminology that has already been revised.
I appreciated the discussion and wanted address some of the specific comments. And for context, here is the original post:
What is the distinction between direct and indirect effects?
The precise definitions of direct/indirect effects have been fuzzy, so one can find a number of different uses in the recent literature. I am partial to the definition and though experiments of direct effects in [Veller et al. (2023)]2 and the sketch of indirect/interpersonal effects in [Benjamin et al. (2024)]3: Direct effects are the average influence of genetic variation in an individual on their own phenotype (possibly mediated by environments). Indirect effects are the influence of genetic variation in an individual on some other person’s phenotype (possibly mediated by other people). These two scenarios are visualized in the figure below.
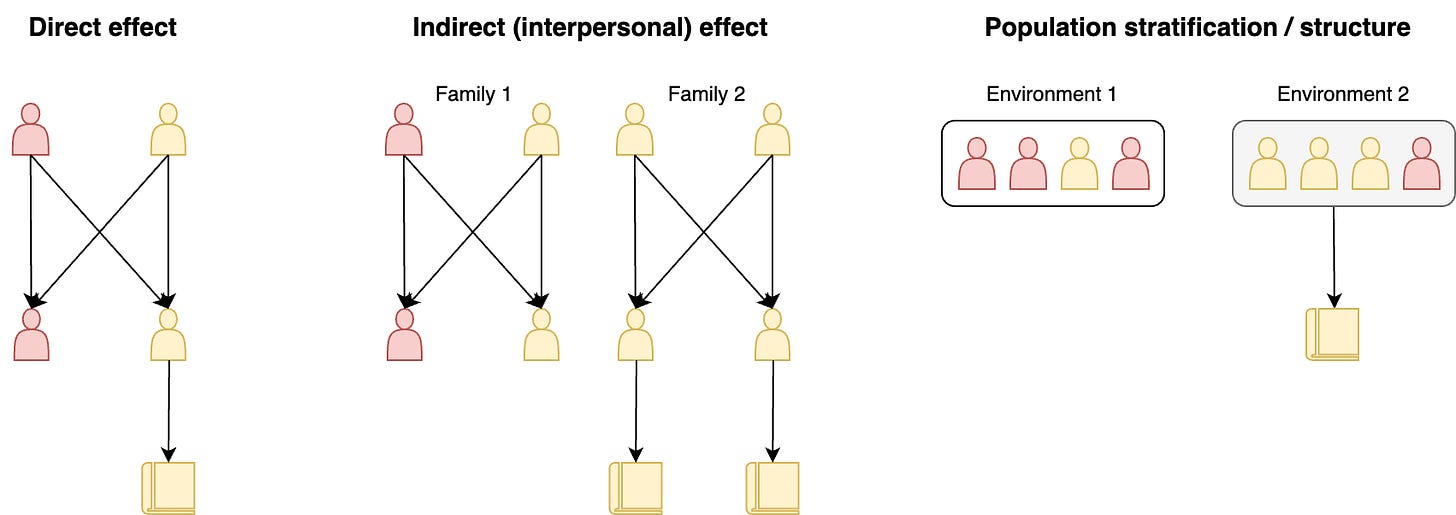
In the left panel, red haired children are forbidden from going to school and blonde haired children are not, so genetic variants that give you blonde hair have a “direct” effect on schooling. In the middle panel, children from families with red-haired parents are forbidden from going to school and children with blonde parents are not. Here the red haired allele in parents is exhibiting an “indirect” effect on the education of their children. Importantly, in this scenario the red hair allele would still be associated with educational attainment in the overall population of children even though it has no direct effect — this is why indirect effects are a confounder. Disentangling direct from indirect effects either requires knowing the underlying mechanism, or contrasting within/between family analyses (more on this later). Finally, in the right panel is an example of stratification: if two populations live in different environments and also exhibit some red hair allele frequency differences due to neutral allelic drift, every single one of the drifted alleles will be correlated with the environment for entirely non-causal reasons. Population stratification, particularly very recent stratification, remains a challenging artifact to account for in genetic analyses.
To emphasize that the type of effect tells us nothing about policy or fairness, consider the following examples of statistically identical direct/indirect genetic effects on educational attainment:
Direct: You have a mutation that alters the function of the DNA repair mechanisms in your neurons and leads to neurodevelopment delays.
Direct: You have a mutation that gives you red hair and you live in a society where red-haired children are forbidden from going to school.
Indirect: You have a mutation that gives you cancer, your son is forced to drop out of college to take care of you. This is a direct effect on your cancer, and an indirect effect on your son’s educational attainment.
Indirect: You are the child of red-haired parents and you live in a society where children of red-haired parents are forbidden from going to school (regardless of their hair color).
It is worth noting that this terminology is not yet settled. Within genomics, papers published this year still use the term “indirect effects” to refer to any genetic associations that are not direct (e.g. Nivard et al. 2024). Outside of genomics, there is the long-standing historic use of “indirect heritability” to imply genetic effects that manifest through the environment rather than “an internal biochemical process”. For example, see Section 4 in Ned Block’s article on heritability and race: if red haired children are forbidden from attending school, the direct effect of a red hair allele is on hair color and the indirect effect is on education. Whereas the definitions above would treat both of these effects as direct because they act within the individual.
How are direct and indirect effects estimated?
The figure above also provides some intuition for how direct effects can be disentangled using genetic data from families. If we measure the red hair allele in pairs of siblings, we can identify siblings that differ in the allele (aka differ “within family”) and quantify how much they differ in their phenotypes. In the case of a direct effect, we would expect to see a difference in educational attainment: the sibling carrying the red hair allele should have lower educational attainment on average. In the case of an indirect effect (e.g. any children of red haired parents cannot go to school) or no effect, we would see no relationship between the allele difference and educational attainment. Conduct the same analysis on every genetic variant and you have what is known as a “sibling GWAS”4, which can then be used to quantify direct heritability.
Isn’t GWAS heritability only quantifying the mechanisms we currently understand?
This is a typical misconception: that GWAS only quantifies the heritability from individual significant associations or genes we understand. In fact, GWAS heritability is defined as the phenotypic variance explained by all genetic variation that has been measured, whether it is significant or not. That said, GWAS still primarily capture all common genetic variants (those with frequency >1%) and typically in Europeans. So GWAS heritability can be interpreted as the total “common variant heritability” within the tested population (but more on this in the next section).
What about the much higher heritability observed in twin studies?
As noted above, the original post focused specifically on contrasting findings from “modern DNA science” for IQ and height, which allows one to make apples-to-apples comparisons within one consistent methodology. A point I also tried to emphasize is that two traits can be fundamentally genetically different even if their population level heritability estimates are similar. A trait like IQ/education appears to be highly culturally and environmentally dependent and behave differently within versus between families or across environments. A trait like height does not. And those differences are far more meaningful than a single heritability parameter. Unlike the analysis of variance, the analysis of individual genetic effects can begin to tell us something about causes, and so far it is telling us that these traits are not alike.
Okay, but why do you think twin estimates so much higher?
Since twin study estimates seem to be such a hangup, allow me to digress a bit and outline why genetic findings also imply that twin studies of behavioral traits should not be taken literally (and maybe not even that seriously).
Broadly speaking there are two ways to estimate heritability. The first, “family-based” approach, is to look at patterns of phenotypic correlations across different relative classes. This approach requires no genetic measurement at all but it makes strong assumptions about the influence of the shared environment and environmental interactions across the relationship classes. The second, “molecular” approach, is to measure genetic variation in unrelated individuals (who are not expected to share environments) and then directly quantify how much phenotype tracks with genotype (using either population or within-family approaches as described above). This approach makes very few assumptions about the environment but it requires all causal genetic variation to be measured. So when there are differences between the two methods, one possible explanation is that the family-based assumptions about environments were inaccurate and the other explanation is that some rare (or otherwise difficult to measure) genetic features haven’t been measured yet. Pretty much every twin researcher acknowledges that the environmental assumptions are likely to be violated to some extent5, and pretty much every molecular researcher acknowledges that genetic variation will be missed to some extent6. There is no perfect study design. So the question becomes which method gets an estimate that is closer to the truth.
In addition to the difference between twin and molecular models, there are substantial differences among twin models for complex behavioral phenotypes like IQ. I’ve written about this in detail previously but the core point is that estimates of heritability tend to be substantially higher in twin models that assume all classes of twins shared their environments equally. In a recent illustrative example, [Bingley et al. (2023)] estimated the heritability of educational attainment to be 41% (with an 18% contribution of the shared environment) using a classic twin model that assumed equal environments for all twins, compared to just 10% (with a 49% contribution of the shared environment) using an extended twin model that relaxed this environmental constraint. In other words, there is not one estimate from twin studies, but many, and they can differ meaningfully.
Because the assumptions of twin models cannot be formally tested and the assumptions of molecular models cannot be tested until all genetic variation is typed, the debate over which model is closer to the truth has persisted for over a decade in various forms (see [Feldman & Ramachandran (2018)], [Gibson (2012)], [Manolio et al. (2009)] and many more). This is the “missing heritability problem” that is one of the motivations for writing this blog.
A substantial breakthrough in this debate arrived with the work of Young et al. (2018), which proposed a new statistical method (Relatedness Disequilibrium Regression, or RDR) that could use molecular data from families to estimate the nearly total direct heritability “without environmental bias” (this statement is literally in the title). I won’t get into the weeds of how this approach works (you can read the paper itself or my technical summary) but the basic idea is to use genetic data from mother/father/child trios to model the full direct and indirect transmission paths. Furthermore, the method was applied in a special genetic dataset from Iceland that had computed the full set of genetic relationships across individuals (known as “Identity By Descent”), rather than just the relationships from common variants measured typically. The one outstanding limitation was that this method could miss extremely rare mutations that occurred after the recent relatedness between pairs of Icelanders (more on this later). What did this approach find?
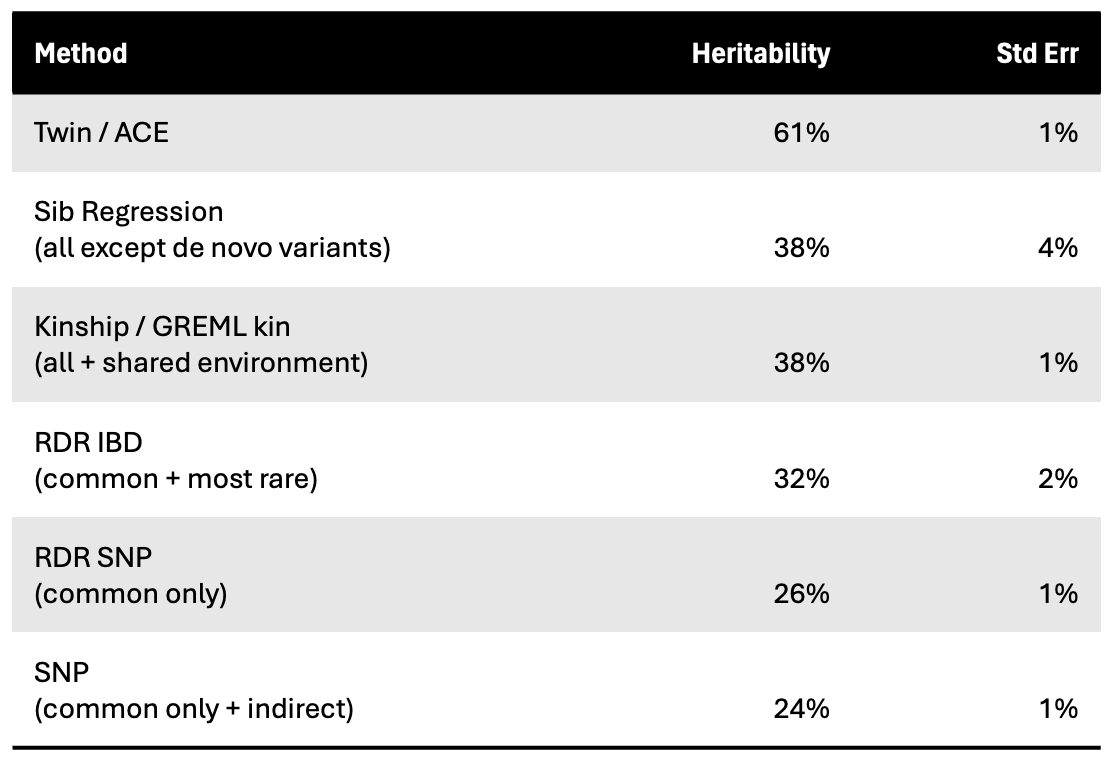
On average across nine traits, the RDR estimate of heritability was 32%, compared to an average twin model estimate of 61% for the same exact traits (though taken from independent registry data). Thus, twin estimates appeared to be ~2x inflated relative to this new estimate of the heritability explained by (nearly) all genetic material. Moreover, an alternative molecular approach called “Sibling Regression” was also applied, which also uses IBD to estimate the heritability of all genetic material including extremely rare mutations RDR could miss (but at the cost of much lower power). This approach had an average heritability estimate of 38% (with wide standard error), slightly higher than RDR but significantly lower than the twin-based estimate. Thus, it was unlikely that ultra rare variants explained the difference between molecular data and twins (the author of RDR has a more detailed argument along these lines). Finally, an application of RDR using only common variant data reached an estimate of 26%, indicating that common variants explained >80% of the overall trait heritability. So the missing heritability problem had largely been solved, and not with a bang but with a whimper: common variants explained most of the heritability and twin study estimates were inflated due to their strict environmental assumptions.
Surprisingly, while these findings made a big splash among geneticists and were seen as essentially a coup de grâce in the heritability debate, the implications — that a century of twin studies had been overestimating their fundamental parameter — were pretty much ignored by the conventional behavioral genetics community itself, where twins have been the methodological workhorse for over a century. To be fair, there were ways to rationalize the ignorance: it’s just one paper, the math is complicated, the standard errors on individual traits were large, the subtleties regarding rare variants and sibling regression were confusing, and the data was not made publicly available — heck, maybe it was all just a bug. Ultimately, what was needed were direct rare variant typing in a very large open dataset.
Fast forward five years and Weiner, Nadig et al. (2023) reported exactly this: a new method (Burden Heritability Regression) for estimating the heritability of rare coding variants and an analysis of 22 traits across hundreds of thousands of individual sequenced exomes from the (widely analyzed) UK Biobank. Coding variants are not all that matters — much of the genome contains functional non-coding elements and it is certainly possible that rare non-coding variants are important too — but if you had to place a bet on where a big chunk of the rare variant heritability was hiding, coding variants would be the first target (as was already the case for low-frequency variants). So what did they find? The average rare coding burden heritability across all traits was just 1.3% (compared to an average common heritability of 13% for the same traits). For fluid IQ, the rare coding heritability was 1.9% (compared to a common heritability of 22%, including indirect effects). In other words, coding burden heritability was expected to add ~10% additional variance explained on top of common variants for the average trait (8% for IQ)7. These independent findings are broadly in line with the expected ~20% contribution from nearly all rare variants estimated by RDR. It is, of course, still possible that there is a massive untapped tranche of ultra rare non-coding heritability that is missed by Sibling Regression and by RDR and by BHR and by direct exome analyses (though, I would argue, not plausible; see the evolutionary modeling in Schoech et al. (2019), for example). But the space of disease architectures that still fit the estimates from twin models is shrinking very fast. Hopefully this bit of history provides some context on why twin studies are not always seen as a high water mark.
What about kinship studies / why do we need to control for relatedness?
Still fixated on heritability estimates, some commenters brought up estimates from kinship-based studies (e.g. Hill et al. (2018)) and also asked why relatedness/kinship needs to be controlled for in GWAS to begin with. These questions have a common answer: individuals that are closely genetically related also tend to share environments, if those shared environments influence their phenotype, then their genetic similarity becomes artificially correlated with phenotypic similarity. In GWAS, this leads to inflation of the test statistic and false-positive associations. In kinship-based heritability estimation, this leads to inflation of the heritability estimate itself8. In the presence of indirect genetic effects, the inflation is even higher because the “environmental” influence is coming from parents/relatives who also directly share genes. In principle, one could include and model genetic information on the parents of every single study participant and … that would be the Relatedness Disequilibrium Regression method described above.
None of these observations are new or controversial. The theoretical point that genetic and cultural transmission can be indistinguishable was made in Cloninger et al. (1979). That specific kinship-based estimates of heritability are confounded by shared environment was then demonstrated in real data in Zaitlen et al. (2013), which also noted likely inflation in twin studies9. This point was again reiterated with extensive simulations in the RDR paper, and discussed in a corresponding blog post10 by the lead author, Alex Young. Alex also regularly has to explain this point on Twitter (sometimes to the same people years apart):
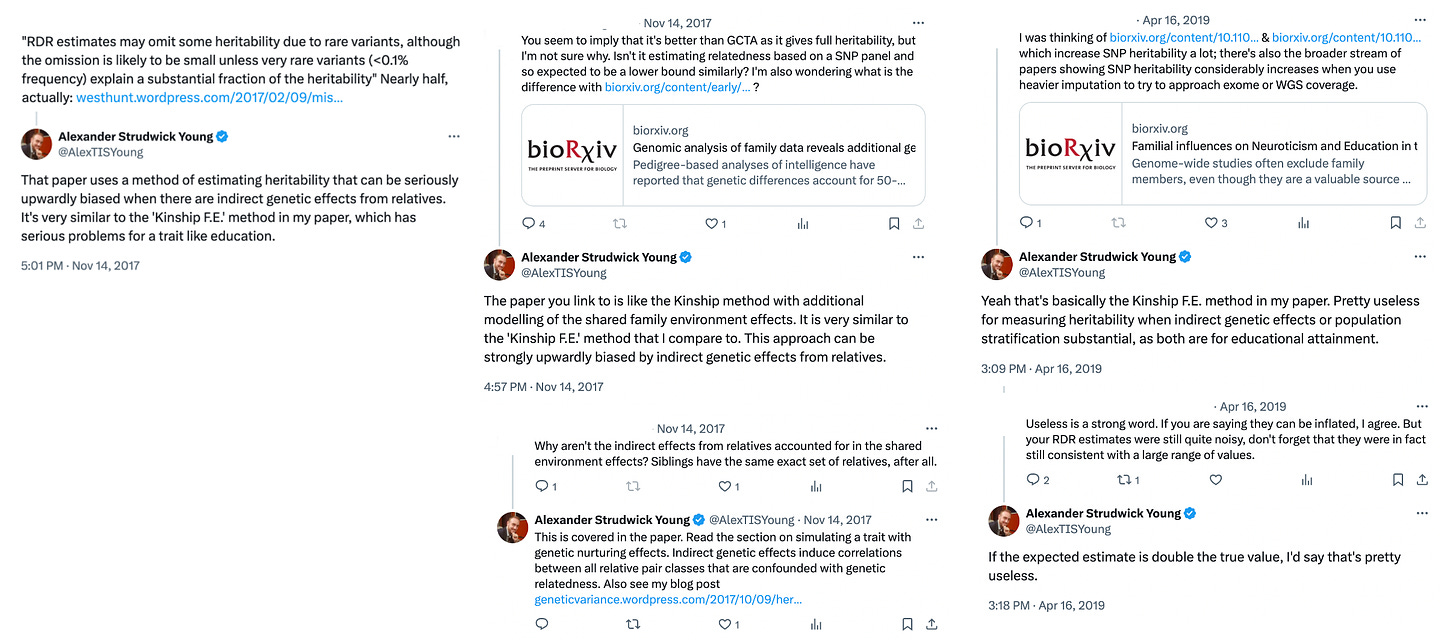
I’m belaboring the examples here to emphasize that people seem to really struggle with the concept that “smart parents have smart kids” is neither necessary nor sufficient evidence of genetic transmission. I’m still optimistic that this understanding will eventually break through but, I’ll be honest, it’s 2024 and seeing the exact same comments Alex was getting in 2017 come up yet again was pretty bleak.
What about higher prediction accuracy reported elsewhere?
Several commenters cited promises of accurate IQ prediction made by Plomin and Von Strumm in their 2018 review “The new genetics of intelligence”. The short answer is that — how should I put this — Robert Plomin tends to make shit up (or, as cognitive scientist Scott Barry Kaufman stated it more delicately: “many of his statements have been riddled with contradictions and logical non sequiturs, and some of his more exaggerated rhetoric is even potentially dangerous if actually applied to educational selection”). In the 2018 review, Plomin claimed that “the EA3 GPS11 is expected to predict more than 10% of the variance in intelligence” based on unpublished data from the 3rd wave of the Educational Attainment GWAS. Citing unpublished findings in a review paper is an odd thing to do in and of itself, but if you do so you should probably make sure the estimates are right. So what did the EA3 GWAS actually show? A prediction accuracy for Total Cognition of 2.7% and for Verbal Cognition of 4.7% (Table S38); nearly identical to the “2-5%” I cited from the IQ GWAS of Savage et al. published the same year (and note, these are all population-level predictors that include indirect effects). To be maximally fair, secondary analyses in the paper were able to combine scores for multiple different traits and eventually get to a prediction accuracy of 9.7% for Cognitive Performance in one of the target cohorts (6.9% in the other). But these numbers still emphasize the core point: even after aggregating many different scores for related traits and cherry-picking the best target cohort, the population-level predictive ability for IQ (9.7%) still remains much lower than that of height (45%). The variability of different predictors and target populations is also why it is far preferable to reason about GWAS heritability: the upper limit on the predictive accuracy of a GWAS-based polygenic score.
What about measurement error?
The measurement error of IQ is a bit of a philosophical question: if we do not know what we are measuring, how do we distinguish error from true variability? Setting aside philosophy, one way error can be quantified is through “test-retest reliability”, where the same individual is assessed multiple times and their results compared12. Most of the reported IQ GWAS use data from the UK Biobank (or similar biobank cohorts) and, lucky for us, Fawns-Ritchie and Deary (2020) retested a subset of these individuals. The resulting test-retest correlation was 0.82 for the general factor of intelligence, which is typically considered high. There also does not appear to be much room for improvement from the perspective of genetics. Williams et al. (2023) investigated a variety of approaches for constructing a high-quality general factor in the UK Biobank, but when the resulting general factor was compared to a single short-form fluid IQ test, both had nearly identical heritabilities (0.201 vs 0.199) and a genetic correlation of 0.93. This is consistent with variability in the test reflecting true variation rather than measurement error. Whatever the explanation, there is no evidence that longer/more thorough IQ tests will substantially change the genetic landscape.
There was something amusing about one of the highest rated comments being a summary of my post that, “to increase uptake”, removed all of the very mild critiques of race science. I guess cognitive decoupling is not so easy after all (though I appreciate whoever went through the trouble).
if we calculate the expected difference in an offspring’s phenotype caused by choosing a gamete at random and flipping its allele, polarizing the difference by the allele that we flip, we obtain [the average direct effect] ~ Veller et al.
When we use the term “genetic effect,” we mean what we have been discussing so far: the effect of an individual’s genotype on that same individual’s phenotype. However, we sometimes instead call it a self genetic effect to distinguish it from an interpersonal genetic effect: the effect of an individual’s genotype on someone else’s phenotype. For example, a parent’s genotype may influence their child’s educational attainment, for example by affecting the parent’s nurturing behavior or income. In the literature, what we call self genetic effects are sometimes called “direct genetic” effects, and what we call interpersonal genetic effects are variously called “indirect genetic,” “associative,” or “genetic nurture” effects. ~ Benjamin et al.
A subtle but important point recently made by [Veller et al. (2023)], is that sibling GWAS provides an estimate of the direct effect that should be interpreted as “locally” causal. Because sibling GWAS requires siblings to differ on the tested allele, their parents must be heterozygous, thus constraining who is being evaluated in the analysis. If heterozygous parents differ substantially on their environments from the rest of the population (and this can happen for a variety of reasons), then the direct effect estimate will only average across this limited set of environments. In the language of causal inference, we can think of heterozygous parents as “compliers” in a randomized trial and the sibling GWAS estimate as the “local average treatment effect”.
However, because estimating heritability coefficients from twin studies and other family studies in humans relies on strong additional assumptions, there has been debate about how well heritability coefficients estimated in humans capture causal associations. … Regardless of debates about the assumptions and ultimate usefulness of estimating heritability coefficients in humans, the finding from twin (and adoption and family) studies—that genetic differences are correlated with (and might or might not be causing) at least some portion of the phenotypic differences—is so basic that Turkheimer enshrined it as The First Law of Behavioral Genetics: “All behavior is heritable.” ~ Hastings Center Reports: Wrestling with Social and Behavioral Genomics: Risks, Potential Benefits, and Ethical Responsibility
Although rare variants explain relatively little heritability, rare variant association studies may still identify variants of large effect that reveal interesting biology and actionable drug targets. On the other hand, rare variants will likely play only a limited role in polygenic risk prediction, which will be largely driven by common variants. ~ Schoech et al. (2019)
And if you are bothered by the assumptions of burden heritability models, these estimates were further supported by an actual rare burden analysis of multiple cognitive phenotypes in the UK Biobank, which identified a mere four genes for IQ (what they call “VNR”) explaining just 0.0015 of the phenotypic variance. I emphasize a focus on heritability estimates rather than counting up association results as the latter is highly dependent on sample size (and the former suggests that there are surely some more IQ genes to be found with larger samples). But 485,930 exomes is nothing to sneeze at, and so few genes being identified is at least facially consistent with the low heritability estimate. If this study had uncovered a large number of novel genes we would need to go back to the drawing board regarding burden heritability. But it didn’t.
In fact, the way GWAS has addressed this issue is by conditioning out all of the kinship heritability into a “random effect” term that is just treated as confounding.
Most previous estimates of heritability are derived from family-based approaches such as twin studies, which may be biased upwards by epistatic interactions or shared environment. Our estimates of heritability, based on both closely and distantly related pairs of individuals, are significantly lower than those from previous studies. We examine phenotypic correlations across a range of relationships, from siblings to first cousins, and find that the excess phenotypic correlation in these related individuals is predominantly due to shared environment as opposed to dominance or epistasis. ~ Zaitlen et al.
We found evidence that GREML methods are likely to have overestimated heritability by around 70%, consistent with the estimated magnitude of genetic nurturing effects. We found evidence that the Kinship method has greatly overstated the heritability of educational attainment, suggesting that a recent study employing a variant of the Kinship method may also have overstated the heritability of educational attainment (see Hill et al., 2018). ~ Young (2023)
“GPS” is the branding for polygenic scores that Plomin and company tried to make stick, presumably alluding to the Global Positioning System that provides accurate estimates for maps and navigation.
Just to make the philosophical argument clear: imagine we are measuring someone’s height in a pitch black room and the person is periodically sitting down or standing up. The “test-retest reliability” of this measurement would not reflect measurement error because the thing being measured is actually changing.
Thank you. I recently started a graph to keep track of the failures and propaganda of the Eugenics movement, and I'm glad to have your article in there. This will grow, and I plan to always have the product to share as I fill it in.
https://embed.kumu.io/8ef99af0bd6e6efa398aef8698828a5a
"Robert Plomin tends to make shit up"
Sometimes the truth hurts.