Science fictions are outpacing science facts for polygenic embryo selection
If you thought prediction in adults was complicated, you haven’t seen the half of it!
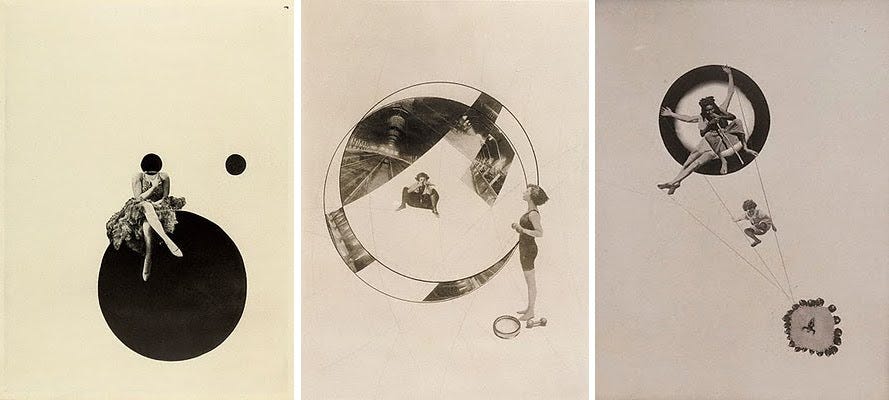
Update: Some additional comments from Shai Carmi (senior author on several papers cited herein).
Last week I wrote about the ineffectiveness of genomic prediction of IQ, motivated by a new direct-to-consumer genetic test. This test is for adults, but much of the response to my post shot off into a discourse black hole on embryo screening and selective breeding. In an effort to provide some clarity, let’s turn to the question of predicting IQ (and other traits) in embryos. If you thought prediction in adults was complicated, you haven’t seen the half of it1!
The basics
As part of the in vitro fertilization (IVF) process, multiple embryos are produced and, traditionally, graded for transfer on various measures of embryo health. Recently, preimplantation genetic testing (PGT) has also been used to predict monogenic (PGT-M) or polygenic (PGT-P) disorders and incorporate genetic prediction into the selection process. With PGT-P a polygenic score is computed for each embryo (just as one would in adults) and either the low-scoring embryos are discarded or the highest scoring embryo is selected. This has opened the door to selecting for a wide range of traits beyond monogenic disorders. Indeed, companies like Orchid are already offering PGT-P screening for schizophrenia, bipolar disorder, Alzheimer’s, cancer, autoimmune disease, and metabolic conditions.
This technology has also generated some controversy (or enthusiasm, depending on your perspective) around the possibility of screening for other behavioral phenotypes. “Polygenic screening permits parents to choose the very best children, according to their own preferences, almost entirely removing the role of luck in the normal genetic lottery” claimed one recent article. In the context of psychiatric traits, Awais Aftab recently wrote an excellent perspective on PGT-P outlining the potential utility and limitations. My goal in this post is not to cover the same ground but to walk through how expected utility is actually calculated and what utility looks like for real behavioral traits. I also want to draw distinctions between the mechanics of PGT-P (polygenic) and PGT-M (monogenic) screening that I think have been under-appreciated. Of course, all of the issues that complicate prediction in adults also apply to embryos.
How and why does it work?
The genetic variance within-family (i.e. between siblings) is half of the genetic variance between families (i.e. in the population). This means that a genetic predictor stratifying across offspring will be half as accurate as in the population. That is a substantial drop in accuracy, but it is also not the complete loss of variance that some may have expected2, and it means that selecting on this variance may have an impact.
Utility is defined by the within-family polygenic score accuracy, since between-family variance is fixed in embryos. Within-family estimates of SNP heritability (e.g. from [Howe et al. 2022]) likewise provide the upper bound on the accuracy of an optimal polygenic score, allowing us to project forward to the expected gains under an optimal (linear) predictor. For most traits the within-/between- family estimates are very similar whereas behavioral, cognitive, and socioeconomic traits often exhibit substantial reductions.
Certain forms of assortative mating will reduce the amount of within-family variance and weaken the power of the predictor. Assortative mating on the phenotype makes offspring more genetically similar to one another and thus reduces the amount of variance available to select from. In contrast, assortative mating on environmental factors (e.g. non-heritable social class) does not impact the genetic similarity of offspring by definition, thus the mechanism of assortment matters. Partner correlations are common for behavioral traits but the mechanism of assortment is disputed.
How well does it work?
Several papers have tackled the expected yield of embryo selection for continuous and dichotomous traits and, interestingly, came to somewhat different conclusions:
In initial work, [Karavani et al. 2019] used breeding theory to derive the expected phenotypic gains for a continuous trait from various embryo selection schemes. They found that selection on continuous phenotypes has limited utility, utility increases slowly with additional available embryos, and they confirmed their theoretical derivations in real data from large families.
In follow-up work, [Lencz et al. 2021] and [Turley et al. 2021] investigated “threshold” traits (i.e. disease traits) and found that while excluding high risk embryos would have little impact on relative risk (<10% currently), selecting the lowest risk embryos could have moderate to large relative risk reductions (close to 50% with some current scores).
I’ll go through each study in turn to to explain how their conclusions are so different, and then talk about some of the additional complexities of polygenic screening.
The utility of selecting for a continuous trait is limited
All you need to quantify the effectiveness of embryo selection is an understanding of within-family segregation variance and the within-family score accuracy or heritability (h2_WF). Then (for example, following derivations in [Lencz et al.]) you can sample a parental mean genetic value with half the variance:
sample embryo values for the i-th embryo, again with half the variance:
sample an environment with the residual variance:
and put them all together to get a phenotype:
I’m belaboring the derivations here a little bit just to convey the fact that this is not a particularly complicated or mysterious process3. [Karavani et al. 2019] have a number of elegant additional results and connections to theory (including deriving the expected gains, conditional expectations, and models of index selection). But simply simulating from the above process is all we need to get some numbers for the main question of utility. When we do so (with 100 families selecting from 10 embryos each and an h2_WF of 0.14) we get something like the figure below: a little bit of genetic variation within the families and a lot of resulting phenotypic variation.

So how well does selecting for a continuous trait work? We’ll use educational attainment and IQ as our model traits, for which the h2_WF was estimated at 4% and 14% respectively in a large-scale recent sibling GWAS [Howe et al. 2022]. I want to underscore that these are the maximum possible accuracies; the current scores [Okbay et al. 2022] are slightly less accurate for education (3.3%) and much less accurate for IQ (4.9%) so I am intentionally estimating the upper bounds on the potential gains from selection: the best one could do with an optimal GWAS-based predictor. The fact that we can know the accuracy of a predictor before we’ve collected the training samples really seems to trip people up but it’s a fundamental aspect of genetic scores.
Let’s plug these values into the equations above and sample 100k families selecting the highest scoring embryo. We’ll use “selection” of a single embryo as the baseline for what one would get with a random draw. The results, shown below, recapitulate the findings from [Karavani et al. 2019] that screening has limited utility for both traits. With 10 embryos, one can get a gain equivalent to ~10 months of schooling if selecting on educational attainment, or 6 IQ points if selecting on IQ scores4. To put those 6 points in perspective, the average 30 day test-retest difference for one individual is 8 points. It goes without saying that this is merely a prediction and the eventual trait has wide variance largely driven by the environment (aka luck), as you can see from the broad distributions.
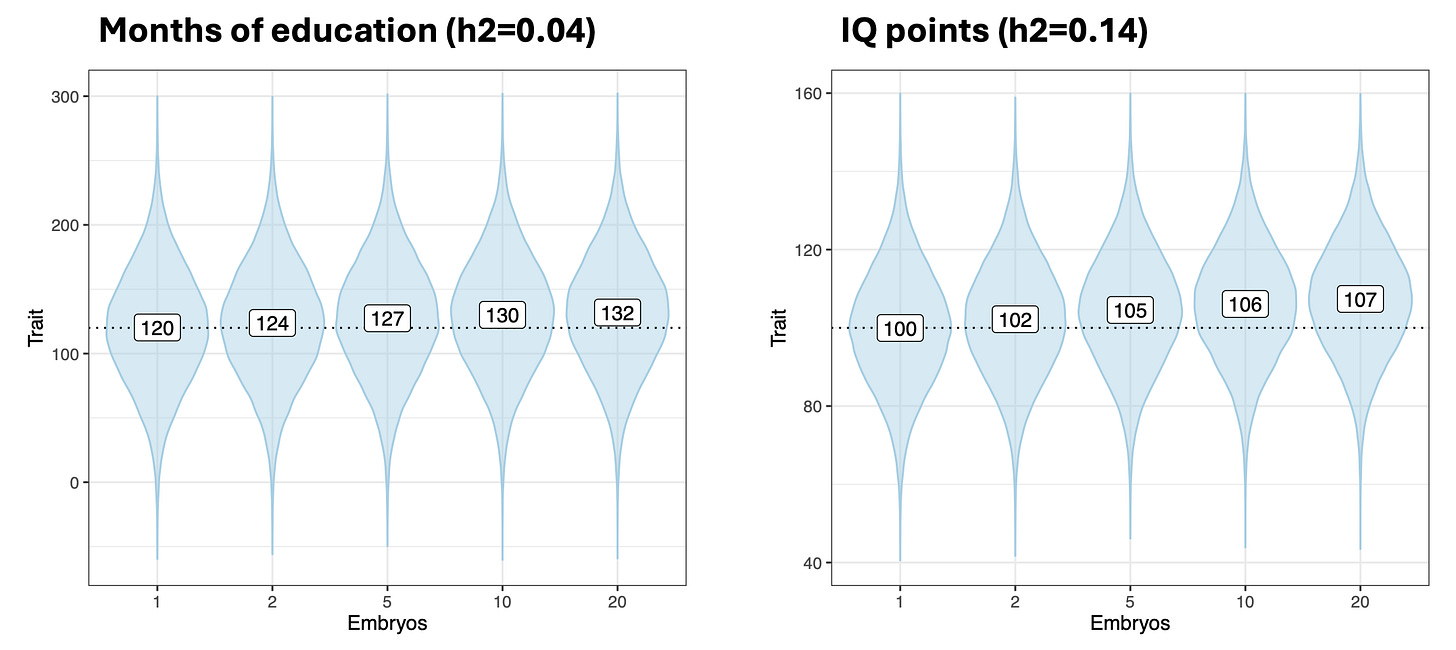
Also as expected, the putative gain quickly plateaus and increasing the number of embryos (in this case to 20) has diminishing returns; there’s only so much within-family variance you can harness. For example, one can gain a predicted ~10 months of schooling by going from 1 to 10 embryos, but for an additional 10 months one would need to increase to a whopping 1,000 embryos. I won’t go into the practicalities of IVF, but suffice it to say that 10 healthy embryos from which to select is already quite optimistic.
In short, embryo selection for cognitive phenotypes is not very effective, and the same principles apply to any other phenotype with low h2_WF. This happens to cover essentially all behavioral phenotypes. Not because behavioral phenotypes are “sensitive” or “controversial”, but because they consistently exhibit low GWAS heritability (e.g. [Cheesman et al. 2017] finding an average SNP h2 of just 0.06 for childhood behavioral phenotypes) and high environmental confounding.
The utility of selecting against a disease depends on the disease model
To understand selection against disease traits, we first need a model of heritable disease. The widely used liability threshold model posits that each individual has an underlying continuous liability (driven by genetic and non-genetic factors) and individuals that cross a given threshold develop the disease (see [Baselmans et al. 2021] for an excellent primer and interactive visualization). The shift from a continuous phenotype to a liability threshold phenotype is visualized in the left and middle panels below. The reason PGT-P can produce substantial relative risk reductions, as noted in [Lencz et al. 2021], is because most disease cases are just above the threshold. Thus nudging them even slightly below the threshold through selection reverts them back to a disease control.
But does nudging someone slightly below the threshold revert them back to a control?
Let’s consider a trait like obesity (one of the traits Orchid screens for) which is defined as having a BMI>40. Using the continuous trait simulations from above, a predictor accuracy of 9% [Khera et al. 2019], and a population SD of 5, we can expect a BMI reduction of about 1 point from 10 embryos (figure below). This is true both in the general population (where BMI drops from a mean of 30 to a mean of 28) as well as in families with BMI>40 even if we completely fix the environment (where BMI drops from a mean of 41 to a mean of 40). Thus we recapitulate the limited utility of embryo selection regardless of baseline risk for BMI.
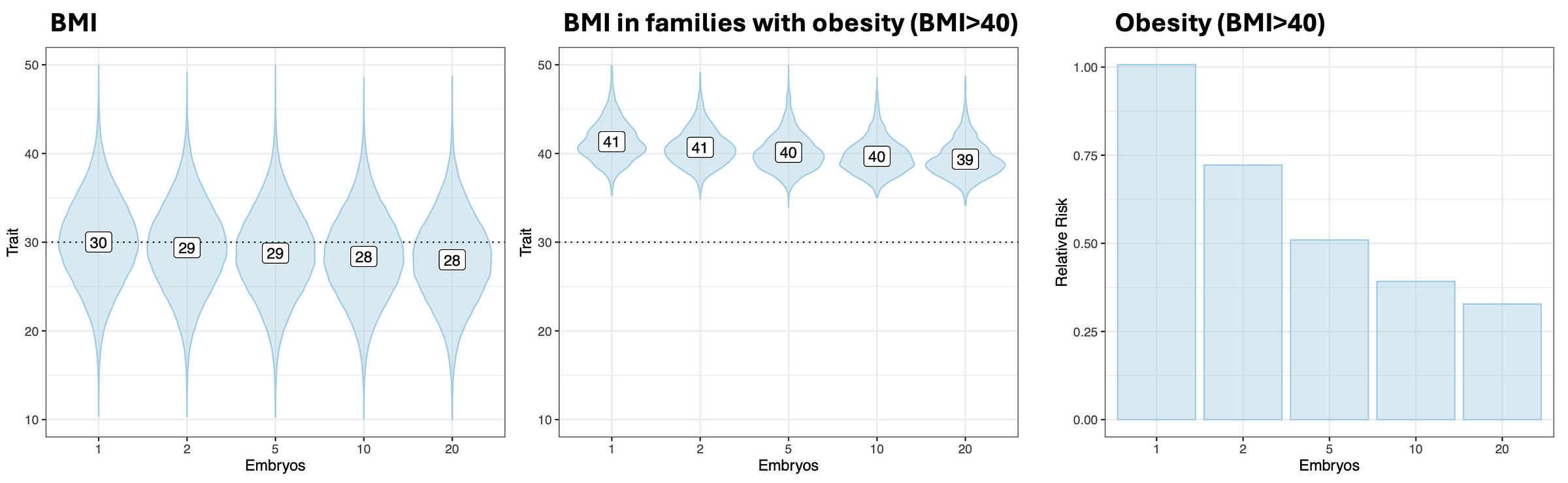
Now let’s reframe this as a threshold trait where individuals with BMI>40 are obese cases and the rest are controls. For simplicity we’ll assume a normally distributed phenotype where ~2% of individuals are obese5. With the same phenotype and the same embryo selection procedure, we now observe a substantial relative risk reduction approaching 45% with 10 embryos (figure above, right). How did this happen? Does setting a threshold wave a magic wand that makes embryo selection much more effective? No, the threshold model simply makes the assumption that reducing someone from BMI 41 to BMI 40 is complete obesity reduction — that they are now below the threshold and therefore can treated as a control. But for a trait like obesity, there’s nothing magical about crossing the BMI 40 threshold; this individual will still have nearly all of the associated health issues.
Alternatively, we can consider a trait like cancer, where — to the best of our understanding — either your cells have transformed into malignant neoplasms that are spreading out of control or they haven’t. If the underlying “threshold” for transformation is at 40 liability “points”, then moving someone down a point from 41 really does reduce their relative risk of cancer: there’s no such thing as half cancer6. The interpretation of relative risk reduction from screening thus depends on the true underlying disease model. For traits like obesity (or IQ for that matter), where the threshold model is diagnostic (doctors need to define the “high risk” cutoff somewhere) rather than mechanistic (a process flips from off to on), substantial risk reduction may be an illusion7.
Finally, let’s consider a disease that follows a “liability spectrum”, where the underlying liability maps to increasing classes of symptom severity, as has sometimes been observed for neurological or psychological conditions. We can define the top 0-1% of the population as definitive “narrow” cases, the next 1-2% as “broad” cases, the next top 2-5% as “mild” cases, and the remaining 95% as completely unaffected. Now we can think about screening through a counterfactual example: imagine you have an offspring and they develop the “narrow” condition, how much could you have reduced their risk by going back in time and using embryo selection to replace them with a different offspring (for simplicity, we will again fix the environment, which would otherwise drive the majority of variation)?
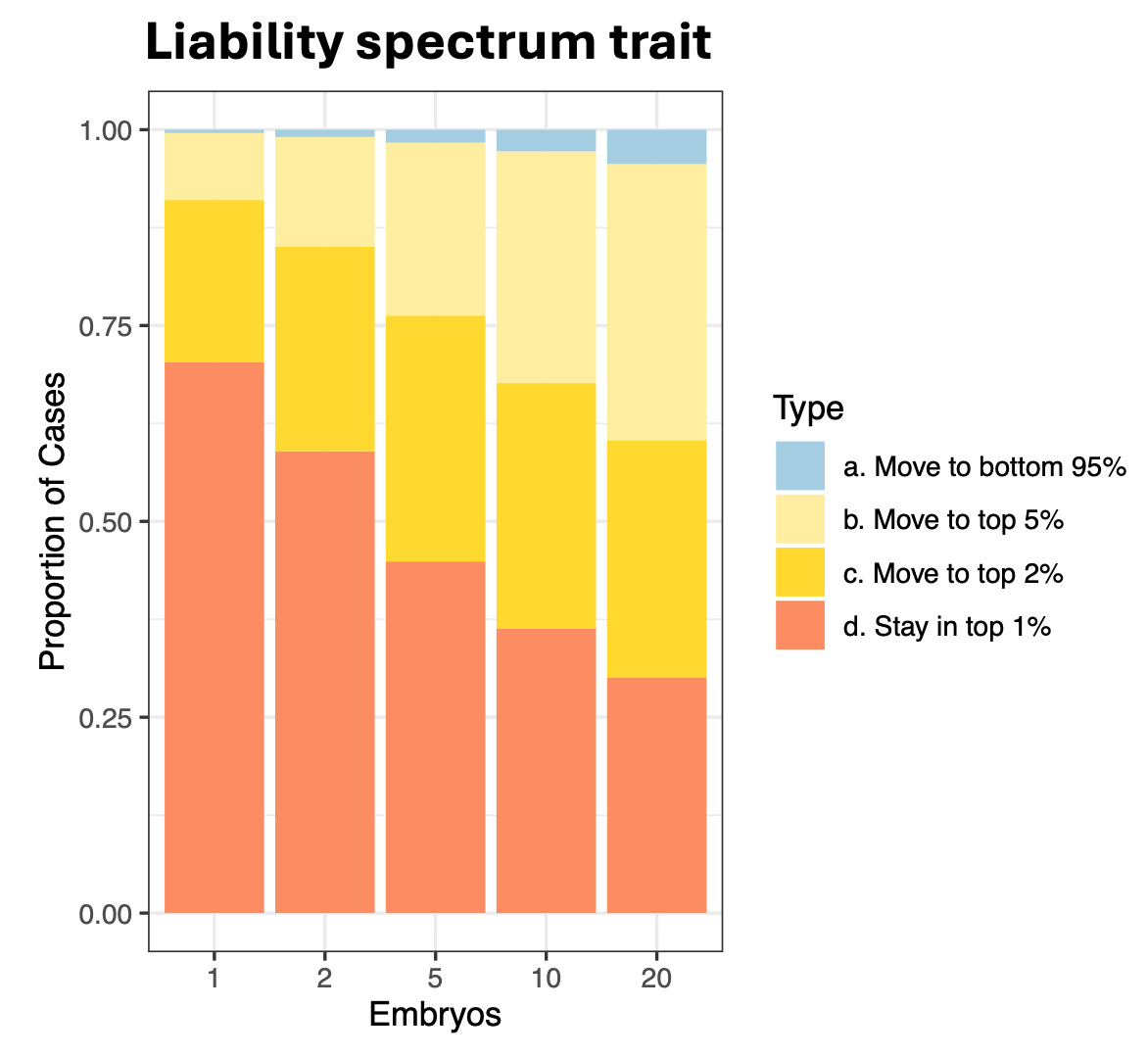
The results of this hypothetical (assuming an h2_WF of 0.08 like the current polygenic score for Schizophrenia) are shown in the figure above and there are several interesting patterns. First, even if you select from a single embryo (i.e. just random selection) the replacement offspring will have some chance (~25%) of no longer being a narrow case. That is, even though the environment is fixed, there is enough within-family genetic variance to shift the liability below the threshold a non-negligible fraction of the time. Second, as you select from a larger number of embryos, the replacement offspring has even lower relative risk of being a narrow case, converging to 0.3-0.4x with 10-20 embryos. This is roughly consistent with the risk reductions we previously observed and that are noted in the literature. Third, the vast majority of replacements who are no longer narrow cases (i.e. below the 1% liability threshold) are still in the top 2-5% of liability; and of those, roughly half are broad cases in the top 2%. Almost no one is replaced with an individual from the bottom 95% of the population. In this example, the consequence of screening is essentially a small reduction of severity rather a reduction of risk.
These dynamics are fundamentally different from screening for monogenic disorders. For Neurofibromatosis (one of many rare diseases that are screened), if you have a homozygous deletion in the NF-1 gene, your cells will not function properly and eventually your nerves will develop tumors; passing down the deletion to your kids will put them at risk for the same. If you have wild-type NF-1, your cells work just fine, you exhibit no symptoms whatsoever, and your kids have baseline risk. For polygenic phenotypes, the entire concept of risk is thus much more contingent on the underlying disease model.
Do neuropsychiatric traits follow a spectrum model?
I’m not a psychiatrist so I will not go deep into nosology, but there is at least some evidence that psychiatric disorders reside on a continuum where risk reduction from cases-just-above-a-threshold to controls-just-below-a-threshold is not the appropriate model. I’ll highlight two examples from the genetics literature:
[Bigdeli et al. 2014] evaluated polygenic risk score values across different diagnostic categories ranging from “narrow” to “broad”. As hypothesized, the “narrow” cases exhibited the highest polygenic score with a monotonic decrease across the milder diagnostic groups. The authors concluded that “the observed pattern of enrichment of molecular indices of schizophrenia risk suggests an underlying, continuous liability distribution”. Ken Kendler, the senior author, has long advocated for a spectrum concept of schizophrenia based on patterns of co-occurrence in families. Under this model, a reduction in genetic liability through screening would lead to a mild reduction in symptoms rather than a reduction in risk.
[Robinson et al. 2016] investigated a continuum of symptoms for Autism Spectrum Disorder (ASD) cases and controls, in a seminal study deploying genetics to inform a neuropsychiatric continuum. ASDs are known to be caused, in part, by a burden of pathogenic de novo mutations. Using a cohort of ASD cases and unaffected siblings, the authors quantified the relationship between this de novo burden and a score for developmental disabilities (the Vineland Adaptive Behavior Scales). The relationship was linear and individuals with the same de novo burden had the same level of social/communication impairment regardless of whether they were cases or controls: “suggesting that the current categorical clinical threshold is largely arbitrary with regard to the social and communication impairments captured by the Vineland” as noted by the authors. These findings were also supported by a more conventional genetic correlation analyses. In this case, a small reduction in the genetic burden through screening would be expected to lead to a small reduction in symptoms.
Paradoxical indirect effects
Now that we understand the published results, let’s talk about two additional complexities. In a previous post I discussed the challenge of indirect/dynastic effects on behavioral traits: there is genetic variation acting in you directly on your phenotype, and there is correlated genetic variation in your relatives that is either acting indirectly on your phenotype or is associated through environmental stratification (both of which appear to be at play for cognitive traits). Such effects can be estimated using genetic data collected from families, where one can partition the variance from offspring, parents, and the covariance of the two. A mystery that has emerged from such family studies is that the two sources of effects are often negatively correlated: the same genetic variation that is associated with increasing the offspring trait by parents (either through parenting or “dynastic” environmental factors) is associated with decreasing the trait in the offspring themselves. In embryo selection, direct effects are the only source of predictive variance for the offspring, but a negative correlation with indirect effects could imply reversion of the trait in subsequent generations. Or, more likely, something complicated about these genetic predictors that we do not understand.
Perhaps the most striking example of negative direct/indirect covariance was observed in the analysis of IQ in the UK Biobank by [Young et al. 2022]:
We also estimated r(δ, α), finding negative correlations (discussed below) for cognitive ability (−0.588, s.e. = 0.094, P = 3.1 × 10−10) and neuroticism (−0.421, s.e. = 0.190, P = 0.027) … If direct effects and NTCs are not very strongly correlated, but both are also correlated with ascertainment, then collider bias could push the correlation estimate in the negative direction and reduce correlations between direct and population effects. Analysis of simulated phenotypes under ascertainment supports this hypothesis, where strong ascertainment reduced r(δ, α) to −0.264 (s.e. = 0.091) for a phenotype with uncorrelated direct effects and parental IGEs (true r(δ, α) = 0)
It is worth working through these results carefully because there is a lot going on. The analysis of IQ identified a highly significant and negative correlation of -0.588 between direct (δ) and indirect effects (here termed α or “non-transmitted coefficients/NTCs”). A hypothesis was proposed that the negative correlation could be a consequence of weak direct/indirect correlations coupled with ascertainment bias. However, a simulation with a direct/indirect correlation of zero and “strong ascertainment” still did not reproduce the magnitude of effects observed in the real data. To state the obvious, a direct/indirect correlation of zero would already be a striking estimate as it implies that the genetic effects on IQ within offspring have no relationship to the family/dynastic effects. And yet, even this unusual baseline coupled with strong ascertainment could not explain the observed results.
This finding is not an outlier or unique to just this one study. Multiple prior analyses of different traits and using different approaches have now observed a similar phenomena:
[Cheeseman et al. 2020] analysis of depression risk in children:
This negative component suggests that genetic variation that is associated with higher depression risk when present in children, has an opposing effect (reducing child depression risk) when present in the parents. On the face of it, this result is difficult to explain and we suggest that it should be interpreted with caution … One hypothesis regarding our finding is that genetic variants that influence mothers to identify depressive symptoms in their children could be linked to emotional sensitivity and may also be associated with parent behaviours that reduce offspring depression symptoms.
[Young et al. 2023] analysis of height and educational attainment:
[For height] if we use the twin estimate of h2_f, we obtain an implausible result that there are IGEs that are negatively correlated with DGEs. This implausible result could be due to overestimation of heritability by twin studies … An alternative (and not mutually exclusive) explanation is that the estimate of r_k is too high.
[For EA] This very high estimate of r_d results in an impossibly high estimate of equilibrium heritability (being statistically significantly above 1) and an inference that there are strong IGEs negatively correlated with DGEs. Two plausible explanations for these implausible results are: overestimation of heritability by twin studies, and confounding in the EA GWAS summary statistics that inflates the correlation between parents’ PGI values.
[Eilertsen et al. 2021] analysis of maternal relationship satisfaction:
The strong positive correlation between maternal and paternal effects may suggest that the same genes contribute to the maternal and paternal effects, whereas the negative correlation between maternal and off-spring genetic effects may indicate that genes have opposing effect when expressed in mothers and offspring
[Bjørndal et al. 2024] analysis of maternal depression:
At 3 years after birth, results indicated that there was a negative gene-environment correlation, for direct genetic and offspring indirect genetic effects. This suggests that the same genes in mothers and offspring work in opposite directions with regards to maternal depressive symptoms at this timepoint.
[Hegemann et al. 2024] analysis of multiple neurodevelopment traits, though simpler models were generally preferred.
In fact, in surveying the literature, negative direct/indirect correlations were observed for cognitive/behavioral traits in most studies where they were tested. These analyses largely focus on parent-child relationship but there is also the question of sibling-sibling effects (i.e. sibling indirect effects), which are almost completely unknown.
I point this out not to argue that genetic variants in parents and children are genuinely exhibiting opposite causal effects (even though some of the above studies do entertain that possibility), but to highlight how little we understand about the genetic and environmental relationships for these traits in general. These are genuinely strange results! It is almost certainly the case that there are sources of confounding in these studies we do not currently have a handle on and which are distorting the resulting polygenic score estimates in unexpected ways and potentially across multiple generations. And since most polygenic scores are currently constructed from population-level GWAS, the scores will contain some unknown mix of direct and (potentially opposing) indirect predictors. Yet again, screening for polygenic traits functions very differently from monogenic screening, where the genetic mechanism is typically clear and non-carriers are otherwise ordinary.
Unknown pleiotropy
In addition to genetic correlations for the same trait across different generations, it is also good to keep in mind that different traits can also be genetically correlated. And because biology is complicated, these correlation can be antagonistic, where increasing a beneficial trait also increases a harmful trait. Educational attainment, for example, is positively genetically correlated with Bipolar Disorder (rg=0.28), Autism Spectrum Disorders (0.30), Anorexia (0.17), and Schizophrenia (0.10), with the mechanisms essentially unknown. IQ is positively genetically correlated with Autism Spectrum Disorders (0.21) and Anorexia (0.06), and negatively genetically correlated with Conscientiousness (-0.25), Extraversion (-0.10), and Agreeableness (-0.10) [Demange et al. 2021]. The latter personality traits are themselves positively correlated with educational attainment, and educational attainment positively correlates with IQ, so even for just this one bundle of phenotypes the relationships are already very complex.
For a correlated threshold trait, the risk reductions we saw above now become risk increases (as pointed out by [Turley et al. 2021] with the specific example of educational attainment and Bipolar Disorder). These effects can be substantial when the correlated trait is more heritable than the trait being selected on. Consider selection on educational attainment (h2_WF = 0.04) which has a genetic correlation of 0.28 with Bipolar Disorder, the latter having a GWAS heritability of 0.20. Selecting on education can unintentionally increase the relative risk of bipolar disorder by ~1.4x (10 embryos). The risk can increase further if the correlated component is even more heritable, as suggested by family studies. Of course, we cannot measure all existing traits nor do we know how truly heritable their correlated components are, so the extent of pleiotropy for any given phenotype under selection is essentially unpredictable.
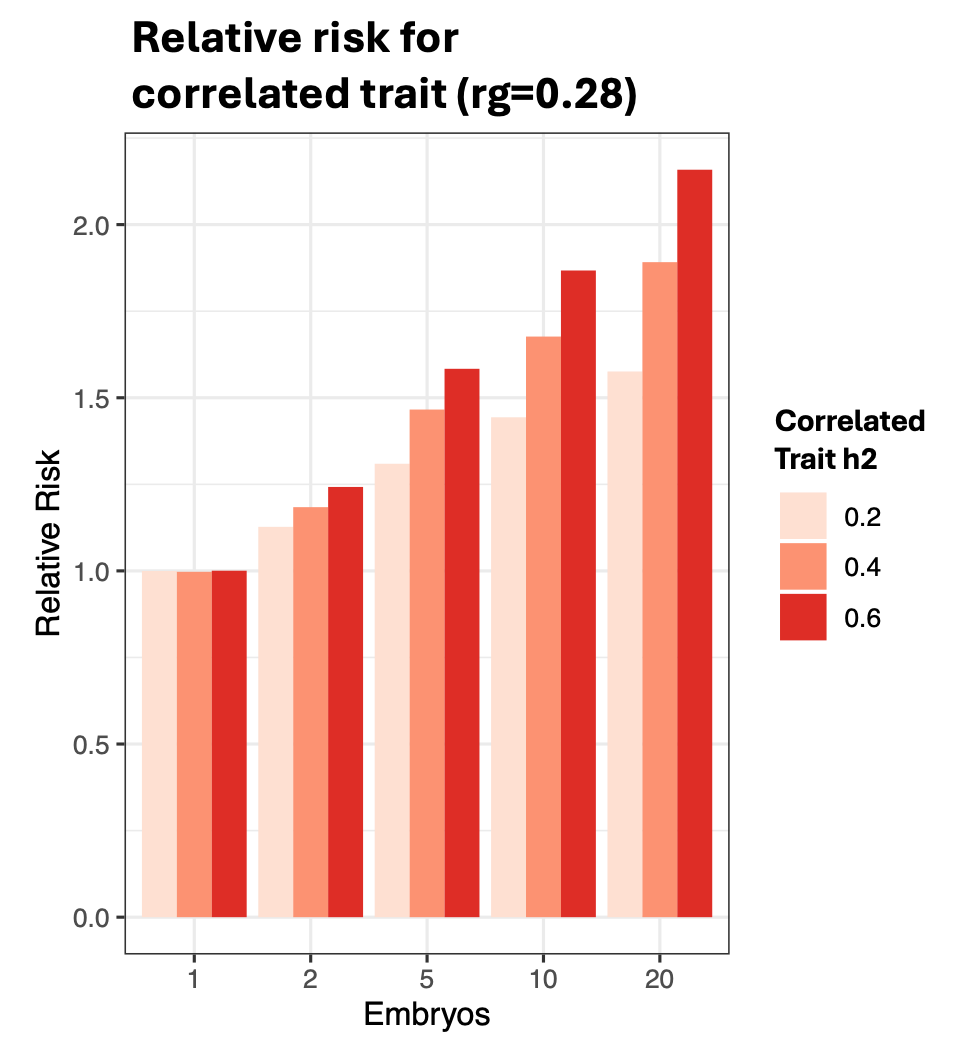
Conclusions and prebuttals
Half of genetic variation is within families, which makes it tempting to think that embryo screening could have meaningful consequences. However, for continuous traits with low within-family heritability — notably cognitive/behavioral traits — the expected gains are minimal even when using an optimal genetic score. The same will be true for disease traits unless they follow a strict biological threshold model. Thus, our expectations should be tempered for disorders that exhibit a spectrum of symptoms or are defined by arbitrary clinical thresholds (as appears to be the case for some neuro/psychiatric conditions like Schizophrenia and Autism). Services promising dramatic risk reductions by slicing continuous traits (BMI) into arbitrary thresholds (obesity) are selling an illusion. Some behavioral phenotypes also exhibit paradoxical negative correlations between transmitted and non-transmitted effects — trait dynamics that we fundamentally do not understand. The resulting predictors will contain more noise than our optimistic simulations, and may have complex multi-generational repercussions. These dynamics are absent from monogenic screening and so the public has no frame of reference through which to understand them.
Finally, the upside of having seen the previous post spiral off into the selective breeding discourse hole is now being able proactively respond to some of the questions that inevitably come up in this discussion, so let’s go through it:
But selective breeding works in agriculture!
[edit: As pointed out in [Karavani et al. 2019], selection in animal breeding generally does not rely on embryo selection but instead leverages the identification of sires from a much larger population as well as shortened generation times.]
I see this response a lot and I genuinely find it baffling. First, the estimated gains above all assume selection works exactly as intended, but they interrogate the utility under realistic parameter values that are relevant to humans. Obviously something can “work” in a technical sense and still produce minuscule yields — which is exactly what we see. Atomic gardening also works well in agriculture, but that tells us very little about its costs and benefits in humans. Second, the concerns raised about model violations fundamentally do not apply to the agricultural setting, where one is free to fix or calibrate the environment. Complex indirect effects, within-family attenuation, the mechanisms of assortative mating, and the spectrum architecture of psychiatric traits — these are factors that are either irrelevant in plants/animals or can be carefully controlled.
Won’t these scores get more accurate as GWAS size increases?
While polygenic scores do increase in accuracy with more training data, the within-family GWAS heritability (h2_WF) is the upper bound on how accurate a GWAS-based score can perform. This is the parameter that was used in the above simulations of educational attainment and IQ.
My friend in Canada has a fancy new algorithm that will be much more accurate.
Hard to argue with this one, but it is at odds with a decade of polygenic score development. Conceptually, most common traits appear to be highly polygenic and driven by very large number of small additive effects: this is the ideal feature space for relatively simple penalized regression models. Recently, [Xu et al.] evaluated a variety of polygenic score methods, including non-linear machine learning approaches, for the prediction of blood cell traits. Blood cell traits are generally sparser and more heritable than other phenotypes and so should be the most amenable to sophisticated machine learning approaches. However, the authors concluded that “the incorporation of nonlinear factors, as in MLP [Multi-layer Perceptron] and CNN [Convolutional Neural Net], did not improve genomic prediction of blood cell traits, compared with linear models” and ended up using Elastic Net, a tried-and-true penalized regression that’s nearly two decades years old. [Rabin et al.] in collaboration with the embryo screening company Genomic Prediction (aka a conflict of interest) investigated a variety of methods and similarly found that old-school penalized regression was best: “LASSO generally performs as well as the best methods, judged by a variety of metrics”. In other words, neither researchers working on basic non-controversial phenotypes nor companies with a vested interest in demonstrating high accuracy are finding any weird tricks to improve score performance.
Won’t these scores become more accurate as the quality of life improves and genetic variation becomes more important?
It is often taken as a given that increasing the quality of the environment will also increase the relative importance of genetics: if environmental variance goes down then the proportion of the trait that is explained by genetics (i.e. the heritability) should go up. At least for cognitive phenotypes, the reality appears to be the opposite: [Mostafavi et al. 2020] estimated the GWAS heritability of educational attainment in individuals grouped by socioeconomic status and found that heritability was lower (and prediction less accurate) in the group with the highest socioeconomic status. [Rask-Andersen et al. 2021] confirmed this result and replicated it for IQ scores and [Cheesman et al. 2022] demonstrated the same for schooling in an independent population. The mechanism is unclear, but one explanation could be that low-SES environments/schools are sink-or-swim, where individuals with small genetically driven cognitive delays are left behind, effectively increasing the influence of genetics. In contrast, in a high-SES environment these individuals are caught up, reducing the role of genetics and the overall heritability. These studies suggest that scores are likely to get less predictive over time if opportunities to catch up are extended to more people.
Shouldn’t you be comparing the highest and lowest scoring embryos?
When we estimate utility, we are comparing between two available actions: select at random (or based on non-genetic factors like embryo health) versus select from the top. It is possible to make embryo selection look slightly more impressive by comparing just the highest scoring and lowest scoring embryos (we could even compare to the lowest scoring embryo in a different family while we’re at it!), but this estimate has no correspondence to a real world action.
IQ is an incredibly valuable trait and correlated with many important outcomes, so any gain is meaningful.
Some people are so fixated on IQ that they can justify any increase no matter how irrelevant. I could shown results with an expected gain 1/100th of a point and there would still be comments about how selection will be worth it in a future where 1 million embryos can be generated or the entire global population is screened or etc. But this is also a fundamental misunderstanding of embryo selection. The relevant question is not whether IQ is correlated with other outcomes, but whether the genetic score for IQ is causal for those outcomes. To spell it out: if there is an environmental confounder (e.g. socioeconomic status) that increases both IQ and, say, income, then IQ will be correlated with income even if the causal genetic relationship harnessed by selection is non-existent.
There are two mechanisms by which selection on IQ can influence an outcome: IQ itself is causal for the outcome, or IQ is genetically correlated within-families with the outcome. With respect to the former, [Davies et al. 2019] estimated the causal effect of IQ on health outcomes using a polygenic-score-like approach called Mendelian Randomization. They found zero effect of IQ on mortality or cancer and minimal effect on other health-related traits: “There was evidence of a total effect of education, but little evidence of a total effect of intelligence on many measures of morbidity and mortality and BMI”. With respect to the latter, [Howe et al. 2022] estimated within-family genetic correlations with educational attainment and found that population-level estimates were substantially attenuated: “There was strong evidence using population estimates that educational attainment is negatively correlated with BMI, ever smoking and CRP. However, these correlations attenuated towards zero when using within-sibship estimates. These attenuations indicate that genetic correlations between educational attainment and these phenotypes from population estimates may be inflated by demographic and indirect genetic effects.”. Thus, IQ appears to neither be particularly causal nor particularly genetically correlated with other valuable outcomes once confounding is addressed8.
So there’s nothing we can do to boost IQ?
As it happens, there is another way to boost IQ and it doesn’t require any genetic testing: education. Multiple genetically informed and non-genetic, quasi-experimental studies have now shown that there is a bidirectional relationship between educational attainment and IQ (estimated in [Davies et al. 2019] but also many others). Notably, the effect of education on IQ appears to be significantly stronger than the reverse. Education itself has some positive direct health benefits and, in contrast to IQ, may even reduce mortality [Howe et al. 2023]. You could say the solution here is a no-brainer!
Further Reading
Turley et al. “Problems with Using Polygenic Scores to Select Embryos”. NEJM. 2021
Capalbo et al. “Screening embryos for polygenic disease risk: a review of epidemiological, clinical, and ethical considerations”. Human Reproduction Update. 2024.
And the associated visualization/calculator.
Karavani et al. “Screening Human Embryos for Polygenic Traits Has Limited Utility”. Cell. 2019
Lencz et al. “Utility of polygenic embryo screening for disease depends on the selection strategy”. eLife. 2021
Yes, this is a joke about the variance in families.
Indeed, some initial criticisms of polygenic embryo screening substantially underestimated the amount of variation within families and argued that it simply could not work at all. This is not an effective line of argument.
This is also to forestall any claims that there exists some hidden/alternative way of doing the calculation that produces very different results.
This is the upper bound. For the current PGS accuracy of 4.9% one would gain ~3.5 IQ points.
In reality BMI follows a log-normal distribution with a heavy right tail and ~10% of the population having BMI>40.
For illustrative purposes, we will ignore phenomena like precancerous lesions, or the fact that even tumors have grade and stage which reflect heterogeneity in the extent of transformation and the severity of cancer spread.
To be clear, the importance of the threshold model assumptions is specifically discussed in [Lencz et al.] and [Turley et al.] (using height as an example). I am raising it here because I think the point has been missed in the context of psychiatric traits.
This also goes both ways: if within-family genetic correlations are low that could imply the risk from unintended pleiotropy may be lower than estimated in the population.
Your substack is outstanding.
“With 10 embryos, one can get a gain equivalent to ~10 months of schooling if selecting on educational attainment”.
Isn’t this huge? In fact, is there any environmental intervention that delivers such a big impact?